Data Analytics Unveiling the Power of Data in Decision-Making
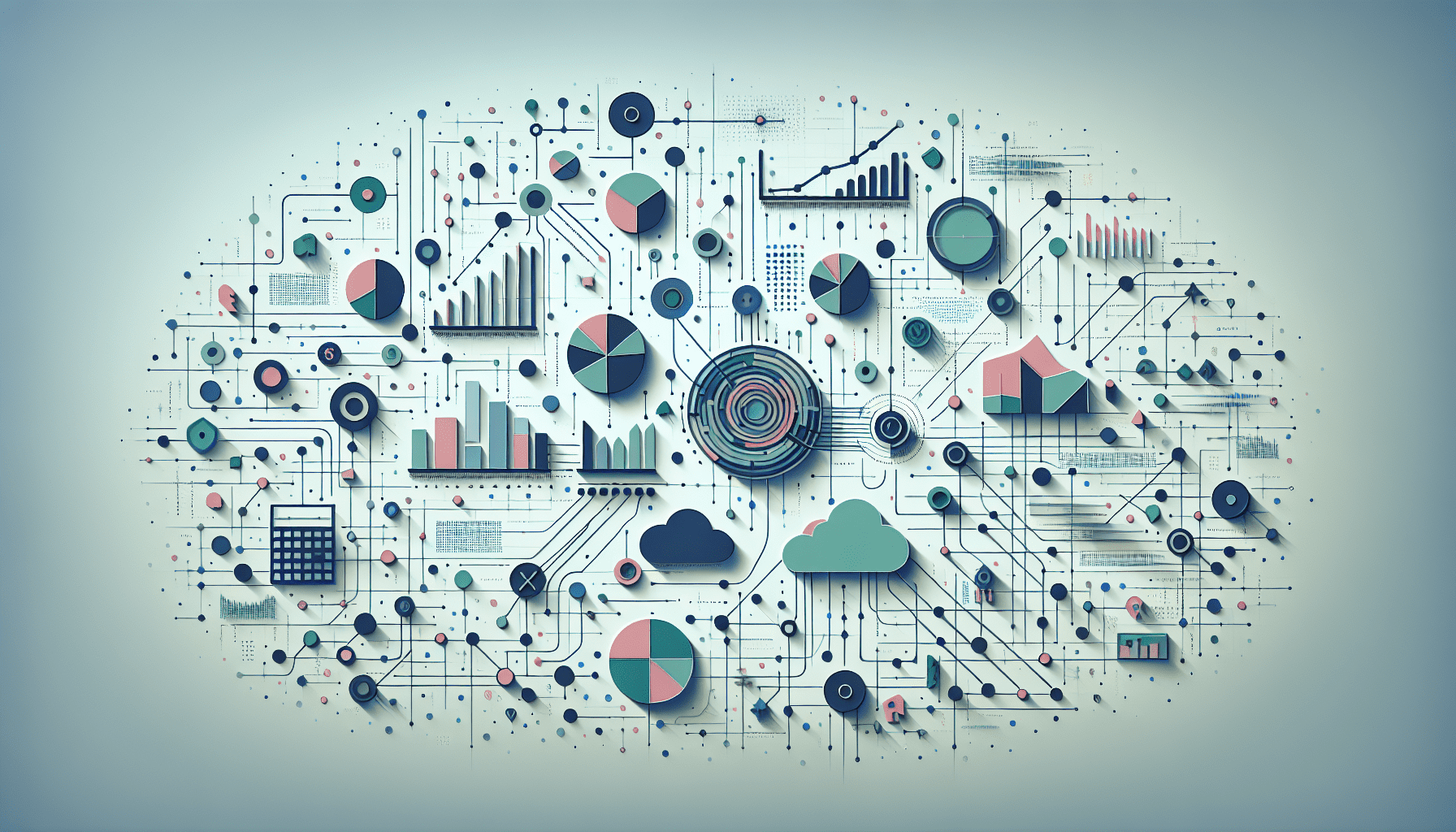
Data Analytics Briefly Summarized
- Data analytics involves the systematic examination of datasets to uncover patterns, extract valuable insights, and support informed decision-making.
- It encompasses a range of techniques including data mining, statistical analysis, predictive modeling, and data visualization.
- The process includes collecting, cleansing, transforming, and modeling data to discover useful information.
- Data analytics is applied across various domains such as business, science, and social sciences to enhance operational effectiveness and scientific research.
- With the advent of big data, data analytics has become a critical component in shaping strategic decisions and driving innovation.
Data analytics is a multifaceted field that lies at the heart of modern decision-making processes. It is the science and art of turning raw data into meaningful insights that can inform strategies, solve complex problems, and create new opportunities. In this comprehensive guide, we will explore the intricacies of data analytics, its techniques, applications, and the impact it has on various industries.
Introduction to Data Analytics
At its core, data analytics is about making sense of data. In a world where data is generated at an unprecedented rate, the ability to analyze and interpret this data has become a valuable skill. Data analytics enables organizations to understand their environment, predict future trends, and make decisions that are based on evidence rather than intuition.
The process of data analytics is iterative and often involves several stages:
- Data Collection: Gathering raw data from various sources.
- Data Cleansing: Removing inaccuracies and inconsistencies to improve data quality.
- Data Transformation: Converting data into a format suitable for analysis.
- Data Modeling: Applying statistical methods to identify patterns or relationships.
- Data Interpretation: Drawing conclusions and making recommendations based on the analysis.
Techniques in Data Analytics
Data analytics employs a variety of techniques, each with its own set of tools and methodologies:
- Descriptive Analytics: This technique focuses on summarizing historical data to identify patterns and relationships. It answers the question, "What has happened?"
- Diagnostic Analytics: Diagnostic analytics delves deeper into data to understand the causes of events and behaviors. It answers the question, "Why did it happen?"
- Predictive Analytics: Predictive analytics uses statistical models and machine learning algorithms to forecast future events. It answers the question, "What is likely to happen?"
- Prescriptive Analytics: This advanced form of analytics suggests actions that can be taken to achieve desired outcomes. It answers the question, "What should we do?"
Applications of Data Analytics
Data analytics finds its application in numerous fields:
- Business: Companies leverage data analytics to optimize operations, enhance customer experience, and increase profitability.
- Healthcare: Analytics can predict disease outbreaks, improve patient care, and manage healthcare costs.
- Finance: Financial institutions use analytics for risk assessment, fraud detection, and portfolio management.
- Sports: Teams analyze player performance and strategize game plans using data analytics.
The Role of Data Visualization
Data visualization is a critical aspect of data analytics. It involves the creation of graphical representations of data, making complex information easier to understand and interpret. Effective visualization helps communicate findings to stakeholders and supports the decision-making process.
Challenges in Data Analytics
Despite its potential, data analytics faces several challenges:
- Data Quality: Poor data quality can lead to inaccurate analysis and misleading conclusions.
- Data Privacy: With increasing concerns over data breaches, ensuring privacy while analyzing data is paramount.
- Skill Gap: There is a growing demand for skilled data analysts who can interpret complex datasets.
Conclusion
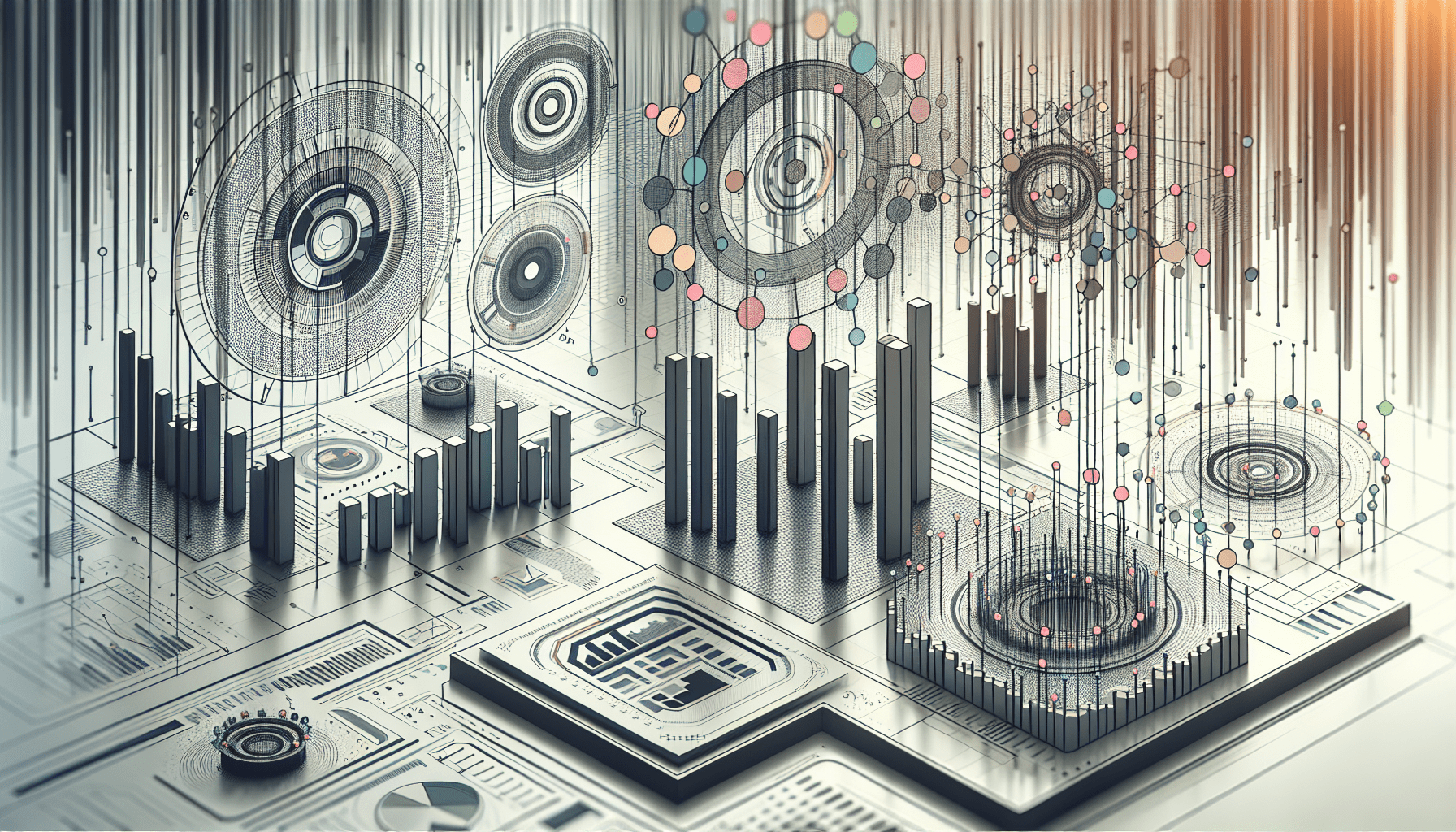
Data analytics is an indispensable tool in the modern data-driven world. It empowers organizations to make informed decisions, predict future trends, and stay competitive. As technology advances, the field of data analytics will continue to evolve, offering even greater insights and opportunities.
FAQs About Data Analytics
Q: What is the difference between data analytics and data analysis? A: Data analysis is a subset of data analytics focused on processing and performing statistical analysis on existing datasets. Data analytics is broader, encompassing the entire lifecycle of data, from collection to analysis to visualization.
Q: What skills are required to become a data analyst? A: A data analyst typically needs strong statistical and analytical skills, proficiency in programming languages like Python or R, and experience with data visualization tools. Knowledge of machine learning and data mining techniques is also beneficial.
Q: How is big data related to data analytics? A: Big data refers to the vast volumes of data generated every second. Data analytics is the process by which this data is analyzed to extract meaningful insights. Big data has significantly expanded the scope and complexity of data analytics.
Q: Can data analytics be automated? A: Certain aspects of data analytics, such as data cleansing and basic analysis, can be automated with the right tools. However, complex analysis and strategic decision-making often require human expertise.
Q: What are the ethical considerations in data analytics? A: Ethical considerations include ensuring data privacy, avoiding data misuse, and preventing biases in data analysis that could lead to unfair conclusions or discrimination.
Sources
- Data analysis
- Data analysis
- Data Analytics: What It Is, How It's Used, and 4 Basic Techniques
- What is Data Analytics? - Definition from WhatIs.com - TechTarget
- What is Data Analytics? | Master's in Data Science
- What is Data Analytics? A Complete Guide for Beginners
- Data Analytics: Definition, Uses, Examples, and More - Coursera
- Google Data Analytics Professional Certificate - Coursera
- What is Data Analytics? A Complete Guide with Demo
- What Is Data Analytics - The Ultimate Guide - CompTIA
- What is data analytics? Transforming data into better decisions - CIO