Understanding the Data Lakehouse Paradigm
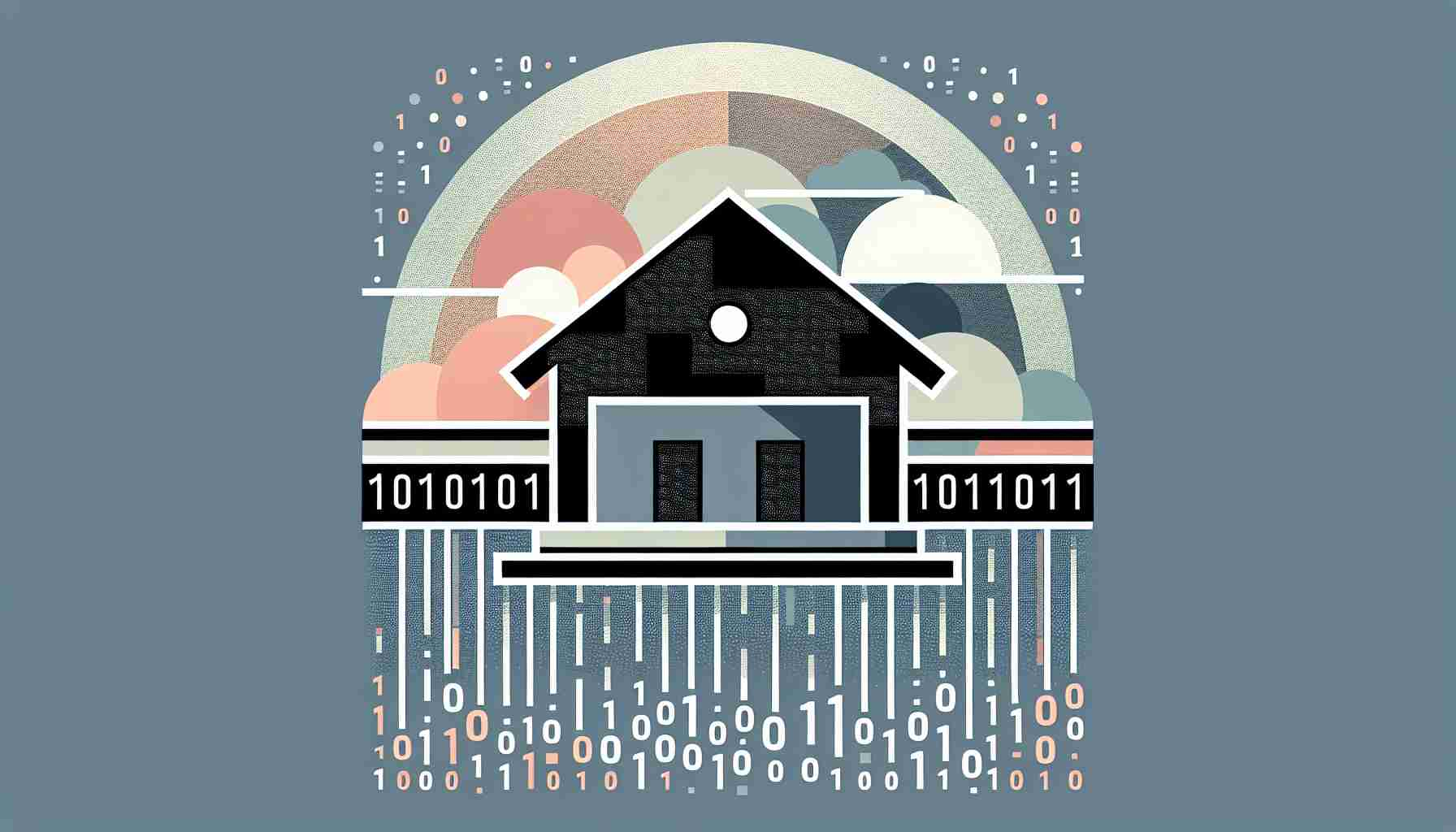
Data Lakehouse Briefly Summarized
- A Data Lakehouse is an innovative data management architecture that merges the expansive storage capabilities of data lakes with the structured querying and analytics power of data warehouses.
- It supports both Business Intelligence (BI) and Machine Learning (ML) operations on a wide variety of data types, including structured, semi-structured, and unstructured data.
- Data Lakehouses are designed to be cost-effective, providing a unified platform for analytical workloads with the flexibility and scalability of modern cloud storage solutions.
- They facilitate a single source of truth for data, integrating storage, processing, governance, sharing, analytics, and AI within one cohesive framework.
- Data Lakehouses can be implemented both on-premises and in the cloud, leveraging services from major cloud providers like Amazon, Microsoft, Oracle, and Google.
The evolution of data storage and management has been a critical aspect of the digital transformation era. As organizations generate and collect vast amounts of data, the need for efficient and scalable data architectures has become paramount. Enter the Data Lakehouse, a term that has been gaining traction in the world of data analytics and big data. This article will delve into what a Data Lakehouse is, its benefits, how it compares to traditional data storage solutions, and its role in the future of data analytics.
Introduction to Data Lakehouse
The concept of a Data Lakehouse represents a convergence of two previously distinct data management paradigms: the data lake and the data warehouse. A data lake is a vast pool of raw data stored in its native format, which can include everything from structured data from relational databases to unstructured data like emails and PDFs. On the other hand, a data warehouse is a repository for structured, filtered data that has already been processed for a specific purpose.
A Data Lakehouse combines the best of both worlds, offering the unstructured data capabilities of a lake with the structured querying and transactional capabilities of a warehouse. This hybrid model aims to provide a single platform that can handle the diverse needs of modern data analytics, including real-time analytics, machine learning, and business intelligence.
Key Components of a Data Lakehouse
Storage and Integration
At its core, a Data Lakehouse is built upon a storage layer that can accommodate a wide variety of data types. This includes structured data in rows and columns, semi-structured data such as CSV files and logs, and unstructured data like documents and multimedia files. The integration component of a Data Lakehouse ensures that data from various sources can be ingested and made accessible in a unified manner.
Processing and Governance
Data processing in a Lakehouse involves transforming raw data into a format suitable for analysis. This can include tasks like data cleaning, aggregation, and enrichment. Governance is another critical aspect, which encompasses data security, quality, cataloging, and lineage. A well-governed Data Lakehouse ensures that data is trustworthy, compliant with regulations, and easily discoverable.
Analytics and AI
The ultimate goal of a Data Lakehouse is to enable advanced analytics and AI. By supporting both BI and ML operations on all data, a Lakehouse empowers organizations to gain deeper insights and drive innovation. The architecture is designed to handle complex analytical workloads, from real-time dashboards to predictive modeling.
Benefits of a Data Lakehouse
- Unified Platform: By consolidating data lakes and warehouses, a Data Lakehouse reduces data silos and simplifies the data architecture.
- Cost-Effectiveness: It leverages the cost benefits of data lakes for storage while providing the analytical capabilities of data warehouses.
- Scalability: Cloud-based Data Lakehouses can easily scale to meet the growing data demands of an organization.
- Flexibility: It supports a wide range of data types and analytical workloads, making it a versatile choice for many use cases.
- Improved Data Quality and Governance: A Lakehouse framework promotes better data management practices, leading to higher quality data.
Data Lakehouse vs. Traditional Data Storage
Traditional data warehouses are optimized for structured data and are not well-suited for handling the volume and variety of data generated today. Data lakes, while capable of storing massive amounts of raw data, often lack the governance and performance needed for complex analytics. The Data Lakehouse architecture is designed to address these limitations by providing a balanced approach that accommodates diverse data and analytical needs.
Implementing a Data Lakehouse
Implementing a Data Lakehouse typically involves selecting a cloud provider or an on-premises solution that supports the Lakehouse architecture. Major cloud providers offer services and tools that facilitate the creation and management of Data Lakehouses. Organizations must also consider aspects like data migration, integration, security, and compliance when adopting a Lakehouse model.
The Future of Data Lakehouse
The Data Lakehouse paradigm is poised to become a cornerstone of data strategy for organizations looking to leverage big data for competitive advantage. As technologies evolve, we can expect to see advancements in the performance, ease of use, and capabilities of Data Lakehouse solutions.
Conclusion
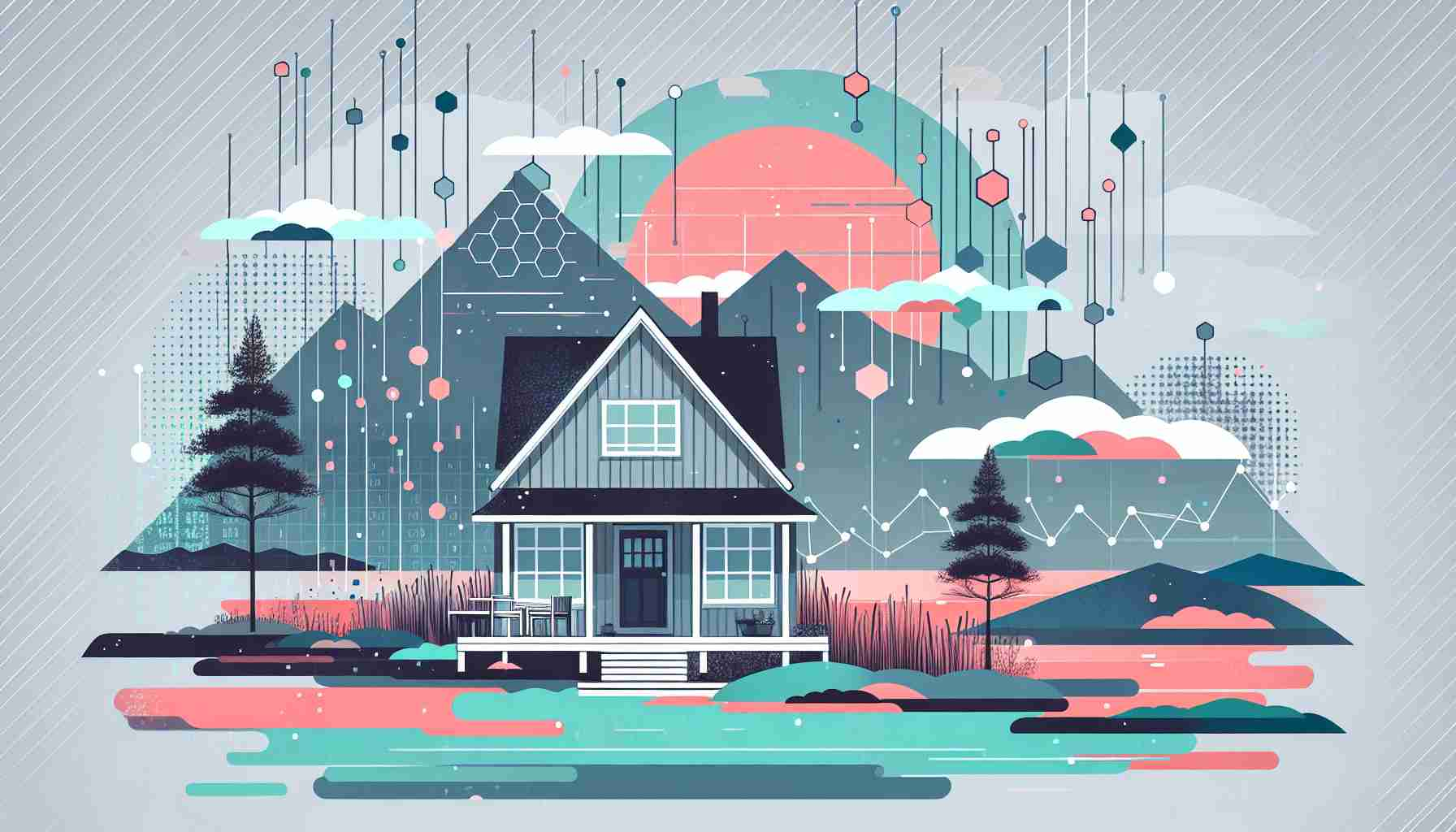
The Data Lakehouse represents a significant step forward in the evolution of data management. By bridging the gap between data lakes and data warehouses, it offers a comprehensive solution that meets the demands of modern data analytics. As businesses continue to navigate the complexities of big data, the Data Lakehouse stands as a promising foundation for data-driven decision-making and innovation.
FAQs about Data Lakehouse
Q: What is a Data Lakehouse? A: A Data Lakehouse is a hybrid data management architecture that combines the storage capabilities of data lakes with the structured querying and analytics power of data warehouses.
Q: How does a Data Lakehouse differ from a data lake or data warehouse? A: Unlike a data lake, a Data Lakehouse provides structured querying capabilities and governance. Compared to a data warehouse, it offers more flexibility in handling various data types and larger volumes of data.
Q: Why is a Data Lakehouse important for AI and ML? A: A Data Lakehouse provides a unified platform that supports the diverse data types and processing requirements needed for AI and ML workloads, enabling more sophisticated and accurate models.
Q: Can a Data Lakehouse be implemented in the cloud? A: Yes, Data Lakehouses can be implemented in the cloud, taking advantage of the scalability and services offered by cloud providers.
Q: What are the challenges of implementing a Data Lakehouse? A: Challenges include data migration, ensuring data quality and governance, integrating various data sources, and managing security and compliance.
Sources
- Data lake
- The Data Lakehouse: The Bedrock for Artificial Intelligence, Machine Learning, and Data Mesh
- What is a Data Lakehouse? - Databricks
- What is a Data Lakehouse? | Glossary | HPE EUROPE
- ¿Qué es un Data Lakehouse? | Técnicas de almacenamiento.
- What is a Data Lakehouse? - IBM
- ¿Qué es Data Lakehouse? | Oracle México
- ¿Qué es un Data Lakehouse? - TurinGears
- Data Lakehouse Architecture - Databricks
- ¿Qué es una instancia de Data LakeHouse? - Azure Databricks