Understanding Data Observability
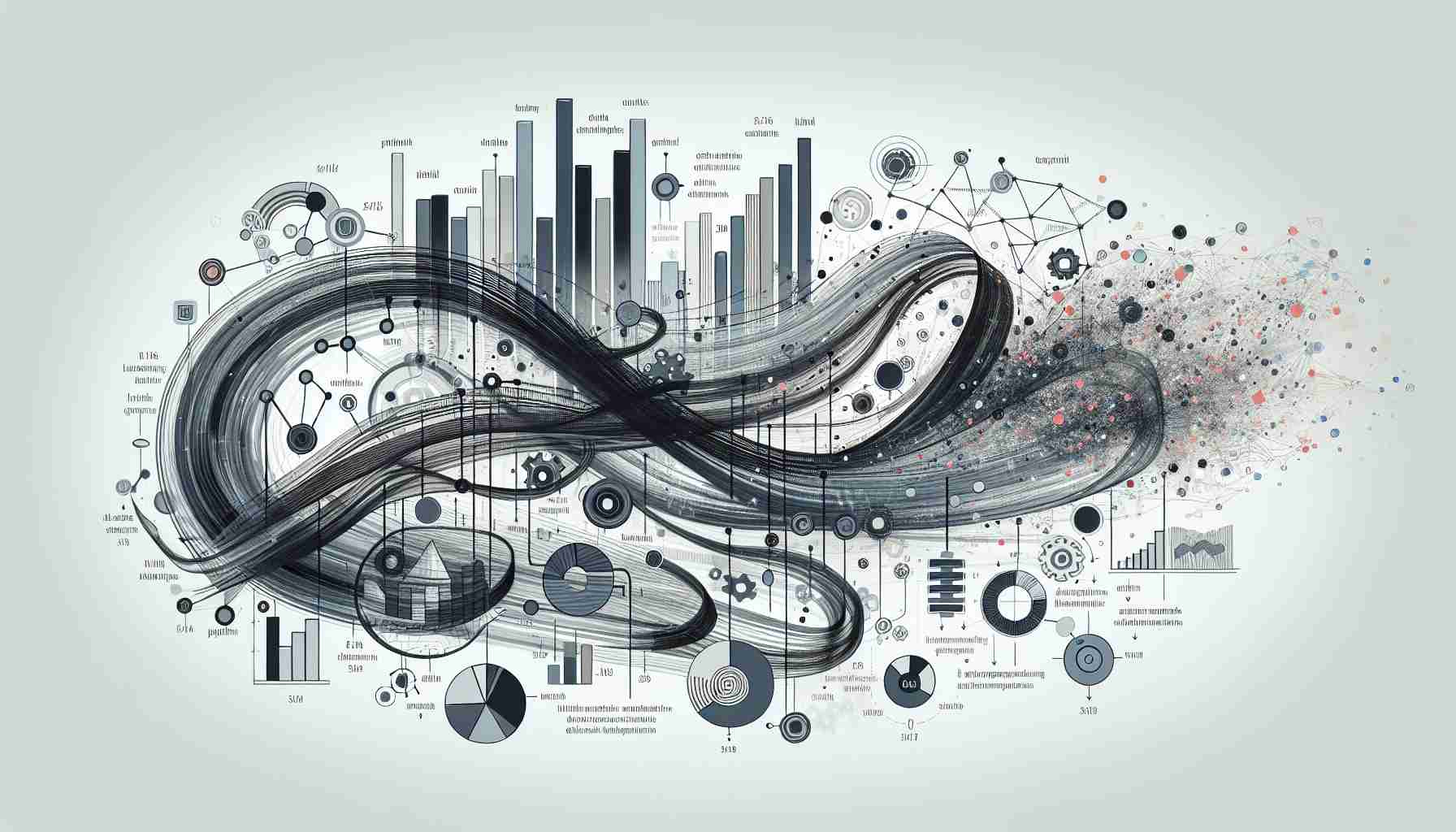
Data Observability Briefly Summarized
- Data observability refers to the ability to fully understand and monitor the health and performance of data within a system.
- It involves tracking data lineage, creating visualizations, and diagnosing issues throughout the data lifecycle.
- Data observability is crucial for maintaining data quality, which includes dimensions such as accuracy and completeness.
- The concept is essential for enterprises to preemptively identify and fix data issues before they impact business operations.
- Observability in data is part of a broader set of practices within DataOps, aimed at improving the overall health of an organization's data systems.
Data observability is a term that has gained significant traction in the realm of data management and analysis. It is a concept that extends beyond the traditional monitoring of systems to provide a comprehensive view of the data's health, quality, and reliability. In this article, we will delve into the intricacies of data observability, its importance, the key pillars that underpin it, and how it is transforming the way organizations handle their data.
Introduction to Data Observability
The term "observability" originates from control theory, where it describes the measure of how well the internal states of a system can be inferred from knowledge of its external outputs. In the context of data, observability takes on a similar meaning but is focused on the ability to understand the state of data flowing through an organization's IT systems.
Data observability is the ability to understand, diagnose, and manage data health across multiple IT tools throughout the data lifecycle. This includes the processes of data ingestion, storage, processing, and analysis. The concept was influenced by the work of Hungarian-American engineer Rudolf E. Kálmán, who introduced the idea of a state observer for linear dynamic systems.
Why Data Observability Matters
In today's data-driven world, organizations rely heavily on data for decision-making, strategic planning, and operational efficiency. However, as data environments grow in complexity, the risk of data issues such as inaccuracies, inconsistencies, and incomplete datasets also increases. These issues can lead to poor decision-making, customer dissatisfaction, and financial losses.
Data observability addresses these challenges by providing a framework for continuous monitoring and understanding of data quality and reliability. It enables organizations to:
- Detect and resolve data issues promptly.
- Ensure high data quality for accurate analytics and reporting.
- Maintain compliance with data governance and regulatory standards.
- Optimize the performance of data pipelines and systems.
The Five Key Pillars of Data Observability
Data observability is often discussed in terms of five key pillars that form the foundation of a robust data observability strategy:
-
Data Freshness: This pillar focuses on the timeliness and relevance of data. It ensures that data is updated regularly and reflects the most current state of the business or process it represents.
-
Data Distribution: This involves understanding the distribution of data values within datasets. It helps in identifying anomalies, outliers, and patterns that could indicate data quality issues.
-
Data Volume: Monitoring changes in data volume can alert teams to issues such as data loss, duplication, or unexpected influxes of data that could affect system performance.
-
Data Lineage: Tracking data lineage involves understanding the flow of data through the system, from its origin to its final destination. This helps in pinpointing the source of data issues and assessing the impact of changes.
-
Data Schema: Observing changes in data schemas is crucial for ensuring that data structures remain consistent and compatible with the systems that use them.
Implementing Data Observability
To implement data observability, organizations must adopt a combination of tools, processes, and cultural practices that promote transparency and proactive monitoring of data health. This includes:
- Utilizing data observability platforms that provide real-time monitoring and alerting capabilities.
- Establishing clear metrics and thresholds for data quality and performance.
- Encouraging collaboration between data engineers, analysts, and business stakeholders to address data issues collectively.
- Integrating data observability practices into the broader DataOps framework to streamline data management and operations.
Conclusion
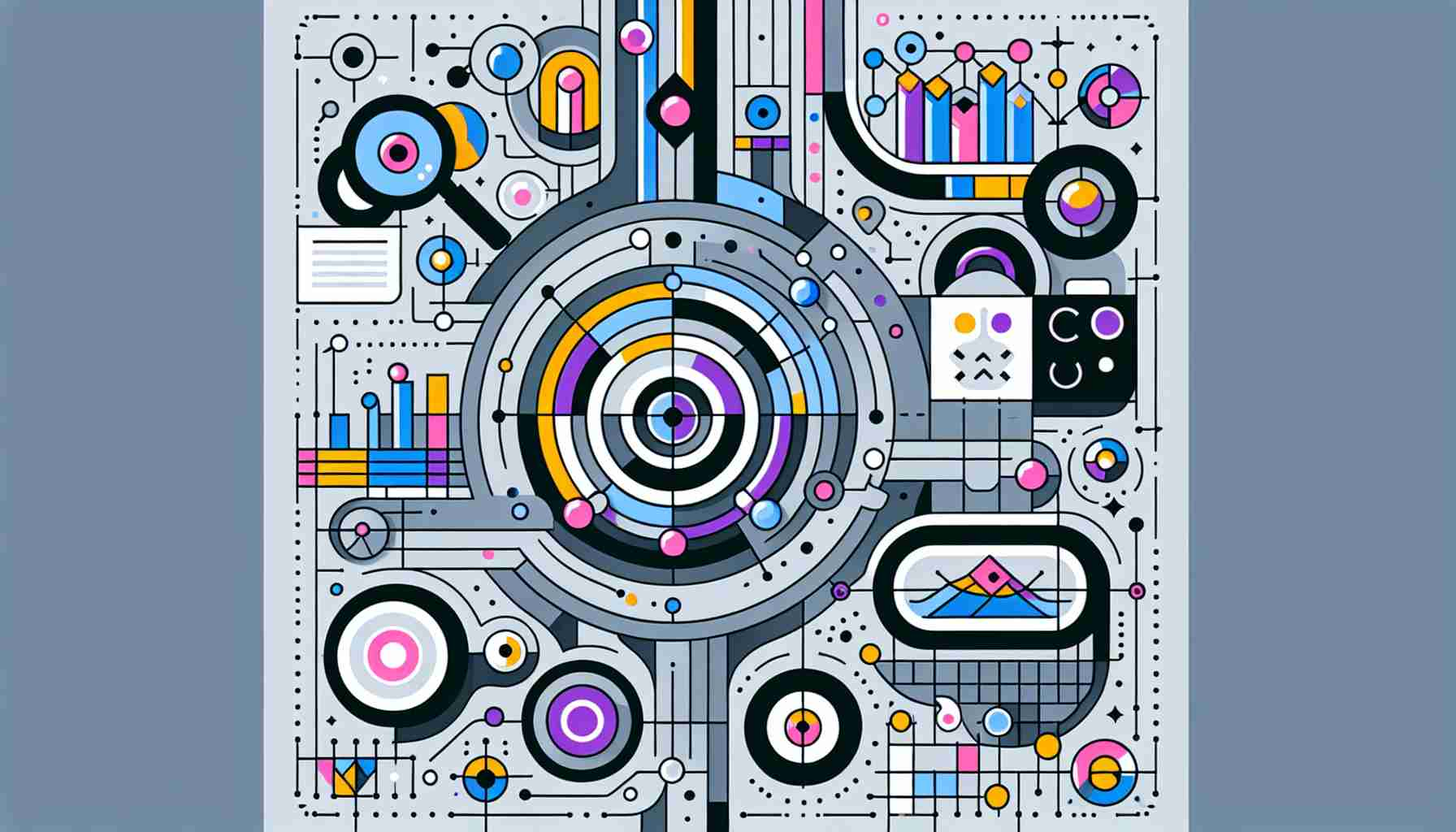
Data observability is a critical component of modern data management strategies. It empowers organizations to maintain control over their data assets and ensures that data remains a reliable foundation for business operations and decision-making. As data environments continue to evolve, the role of data observability will only become more integral to the success of data-driven initiatives.
FAQs on Data Observability
Q: What is data observability? A: Data observability is the ability to understand, monitor, and manage the health and performance of data within an organization's IT systems.
Q: Why is data observability important? A: It is important because it helps organizations maintain data quality, quickly resolve data issues, and make informed decisions based on reliable data.
Q: What are the key pillars of data observability? A: The key pillars include data freshness, data distribution, data volume, data lineage, and data schema.
Q: How does data observability differ from data monitoring? A: Data observability provides a more comprehensive and proactive approach, focusing not just on monitoring but also on understanding and diagnosing data issues.
Q: Can data observability improve data governance? A: Yes, by providing insights into data quality and lineage, data observability can support better data governance practices and regulatory compliance.
Sources
- Observability
- What Is Data Observability? 5 Key Pillars To Know In 2024
- What is Data Observability? All You Need to Know - Acceldata
- Data Observability Explained - StrongDM
- Data Observability: The Complete Introduction - Splunk
- What Is Data Observability and Why You Need It? - Precisely
- What Data Observability Is, What It's Not, and Why it Matters
- What Is Data Observability, and How Will It Help You Succeed?
- What is Data Observability? Why is it Important to DataOps?
- Data Observability and its Importance: Everything you Need to Know