Understanding Data Quality in Data Analysis
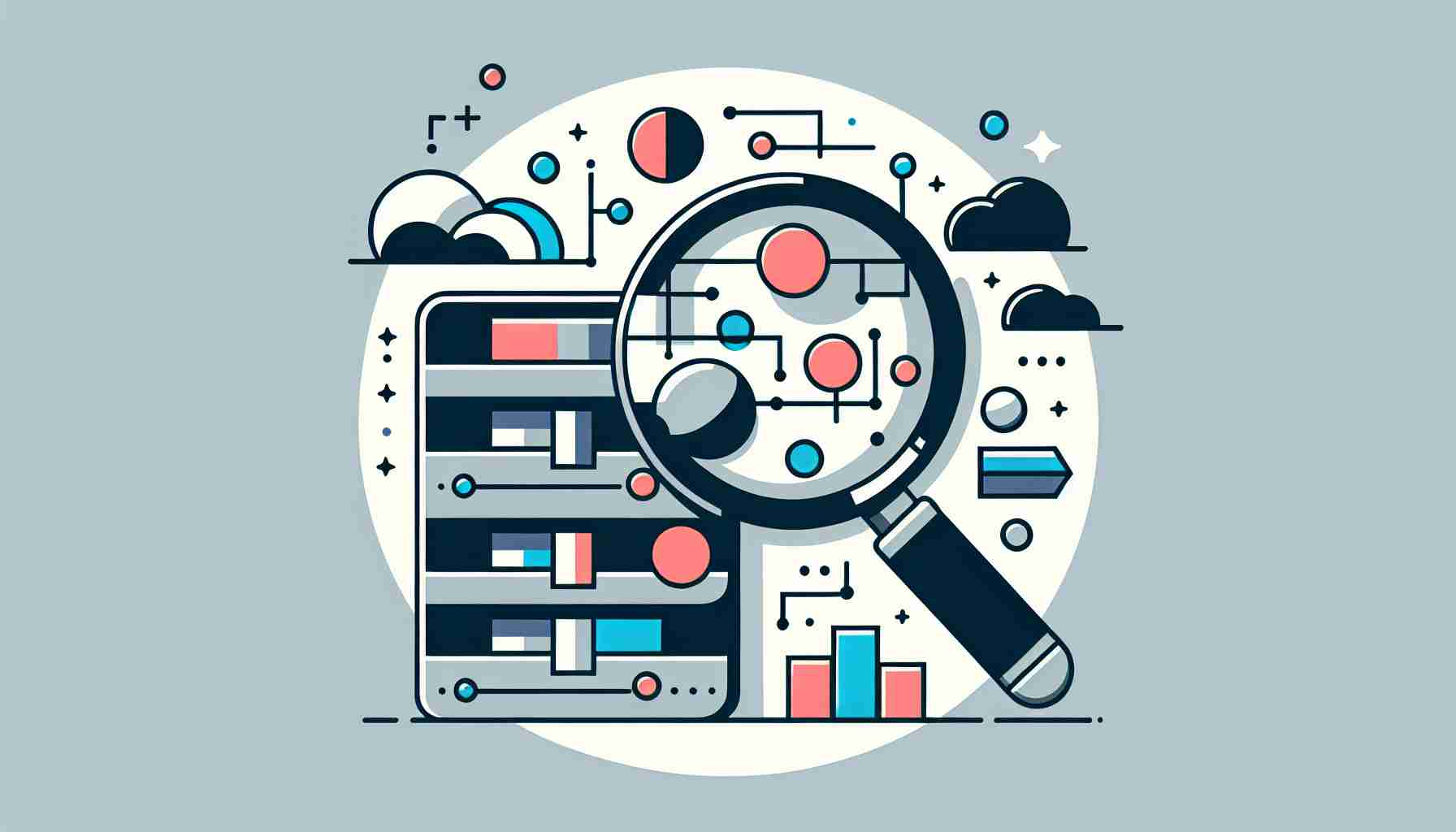
Data Quality Briefly Summarized
- Data quality refers to the condition of data based on attributes such as accuracy, completeness, consistency, and reliability.
- High-quality data is "fit for its intended uses in operations, decision making, and planning" and accurately represents the real-world construct it refers to.
- The dimensions of data quality include accuracy, completeness, validity, consistency, uniqueness, timeliness, and fitness for purpose.
- Data governance plays a crucial role in establishing agreed-upon definitions and standards for data quality.
- Ensuring data quality often requires data cleansing to maintain internal consistency and meet external purposes.
Data quality is a multifaceted concept that sits at the heart of data analysis and decision-making processes. In an era where data is ubiquitous and drives most of the strategic decisions, the importance of data quality cannot be overstated. This article delves into the nuances of data quality, its dimensions, significance, and best practices to maintain it.
Introduction to Data Quality
Data quality is the measure of data's condition that influences its ability to serve its intended purpose in operations, decision-making, and planning. It is a critical aspect of data management and has a direct impact on the analytical outcomes and business intelligence insights derived from data.
High-quality data must correctly represent the real-world constructs to which it refers. It should be noted that different stakeholders may have varying perspectives on what constitutes 'quality' in data, often leading to the need for robust data governance to achieve a common understanding and standards.
Dimensions of Data Quality
To fully grasp what data quality entails, it is essential to understand its dimensions:
- Accuracy: The extent to which data is close to the true values.
- Completeness: The degree to which all required data is available.
- Consistency: The uniformity of data across different datasets and systems.
- Validity: Data that conforms to the specific syntax (format, type, range) and semantics of its definition.
- Uniqueness: Ensuring that each data entry is distinct and not duplicated.
- Timeliness: Data should be up-to-date and available when needed.
- Integrity: The correctness and reliability of data throughout its lifecycle.
These dimensions are not exhaustive but provide a framework for assessing data quality.
The Importance of Data Quality
The significance of data quality is evident across various facets of an organization:
- Decision Making: High-quality data leads to better-informed decisions.
- Compliance: Adherence to data quality standards is often required for regulatory compliance.
- Customer Satisfaction: Accurate data can improve customer interactions and satisfaction.
- Operational Efficiency: Good data quality reduces errors and increases the efficiency of business processes.
Ensuring Data Quality
Maintaining data quality is an ongoing process that involves several best practices:
- Data Governance: Establishing policies and standards for data management.
- Data Profiling: Assessing the data for errors or inconsistencies.
- Data Cleansing: Correcting or removing inaccurate records from a dataset.
- Data Enrichment: Enhancing data quality by adding missing or additional information.
- Continuous Monitoring: Regularly checking data against quality metrics.
Challenges in Maintaining Data Quality
Organizations face several challenges in maintaining data quality, such as:
- Volume of Data: The sheer amount of data can make quality management daunting.
- Data Integration: Combining data from various sources often leads to inconsistencies.
- Human Error: Manual data entry and handling can introduce errors.
- Evolving Data: As business needs change, so do the requirements for data quality.
Conclusion
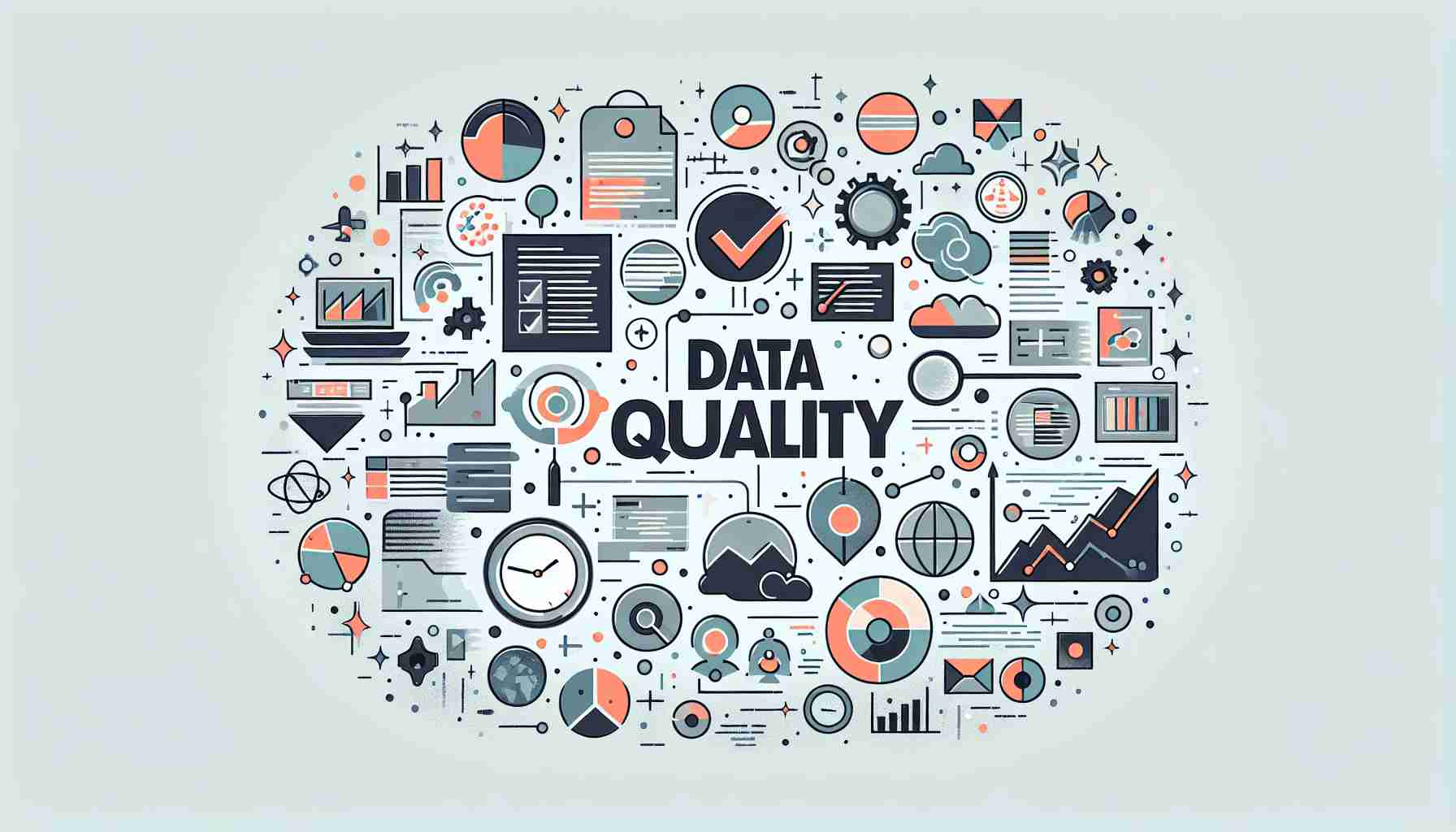
Data quality is a cornerstone of effective data analysis and business intelligence. It requires a strategic approach and commitment from all levels of an organization to ensure that data is accurate, complete, and reliable. By prioritizing data quality, organizations can make more informed decisions, improve customer satisfaction, and maintain a competitive edge.
FAQs on Data Quality
What is data quality? Data quality refers to the condition of data based on attributes such as accuracy, completeness, consistency, and reliability, which determine its suitability for a specific purpose.
Why is data quality important? Data quality is crucial for accurate decision-making, operational efficiency, regulatory compliance, and customer satisfaction.
What are the dimensions of data quality? The dimensions of data quality include accuracy, completeness, validity, consistency, uniqueness, timeliness, and integrity.
How can organizations ensure high data quality? Organizations can ensure high data quality through robust data governance, data profiling, data cleansing, data enrichment, and continuous monitoring.
What challenges do organizations face in maintaining data quality? Challenges include managing large volumes of data, integrating data from various sources, human error, and evolving business requirements.
Sources
- Data quality
- What is data quality? - IBM
- What Is Data Quality and Why Is It Important? - Alation
- Data quality - Wikipedia
- What is Data Quality? Why You Need It & Best Practices - Qlik
- The 6 Data Quality Dimensions with Examples | Collibra
- What is Data Quality? - Informatica
- What Is Data Quality? Dimensions, Benefits, Uses - Dataversity
- What is Data Quality? Definition and FAQs | HEAVY.AI
- What is Data Quality? - TIBCO