Understanding Data Trends in Data Analysis
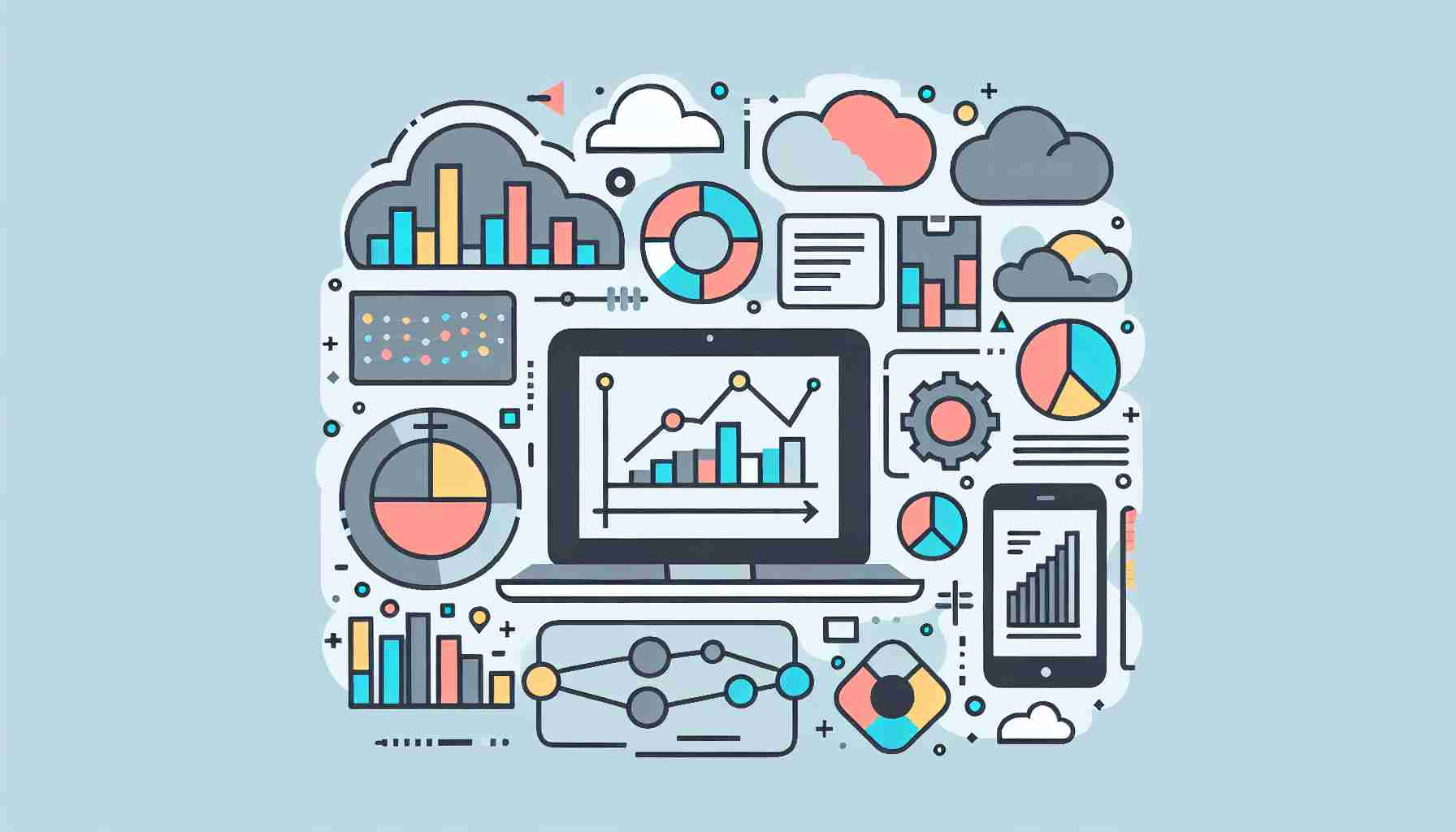
Data Trends Briefly Summarized
- Data trends refer to patterns, changes, and insights derived from data analysis over a certain period.
- They are crucial for businesses and organizations to make informed decisions, predict future scenarios, and stay competitive.
- Technological advancements such as AI, machine learning, and cloud computing are driving the evolution of data trend analysis.
- Tools like Google Trends provide insights into public interest and behavior by analyzing search query volumes.
- Current trends in data analysis include the integration of AI, emphasis on data privacy and security, and the rise of hybrid cloud solutions.
Data trends are the lifeblood of modern data analysis, offering a window into the past, present, and potential future of various aspects of business, technology, and society. By understanding data trends, organizations can gain a competitive edge, adapt to market changes, and innovate effectively. This article delves into the concept of data trends, their importance, the tools used to identify them, and the latest trends in data analysis as we navigate through 2024.
Introduction to Data Trends
Data trends are patterns or movements in data that occur over a period of time. These trends can be upward, downward, or cyclical and are often identified through the use of statistical analysis and visualization techniques. In the context of data analysis, recognizing and understanding these trends is essential for forecasting, strategy development, and decision-making.
The Importance of Data Trends
Data trends are not just about observing what has happened; they are about predicting what could happen next. They enable businesses to anticipate market demands, understand customer behavior, and respond to emerging challenges. By analyzing data trends, companies can optimize their operations, tailor their marketing strategies, and innovate their products and services to meet the evolving needs of their target audience.
Tools for Identifying Data Trends
Several tools and platforms are available to help analysts and businesses identify and monitor data trends. One such tool is Google Trends, which analyzes the popularity of search queries in Google Search across various regions and languages. It provides graphs to compare the search volume of different queries over time, offering insights into public interest and behavior.
In 2008, Google launched Google Insights for Search, which was later merged into Google Trends in 2012. This advanced service displays more sophisticated search trends data, allowing users to explore issues and events in detail, curated by the Trends Data Team.
The Evolution of Data Trends Analysis
The way we analyze data trends has evolved significantly with technological advancements. The integration of artificial intelligence (AI) and machine learning into data analytics has allowed for more accurate predictions and deeper insights. Business intelligence tools have become more sophisticated, enabling organizations to gather insights more efficiently.
Hybrid cloud solutions and cloud computing have also transformed the landscape of data analysis, providing scalable and flexible environments for managing and analyzing large datasets. Data fabrics and metadata-driven approaches are streamlining the way data is accessed and used across organizations.
Current and Emerging Data Trends
As we look towards 2024, several key data analytics trends are shaping the industry:
-
AI-Powered Data Analytics: AI continues to play a pivotal role in data analytics, with machine learning algorithms improving predictive analytics and decision-making processes.
-
Data Privacy and Security: With increasing concerns over data breaches and privacy, organizations are prioritizing the security of their data and the ethical use of analytics.
-
Hybrid Cloud Solutions: The adoption of hybrid cloud environments is on the rise, offering a balance between on-premises, private cloud, and public cloud services.
-
Data Fabric: The concept of data fabric is gaining traction, providing a unified architecture and set of data services that support a wide range of use cases across different platforms.
-
Edge Computing: As the amount of data generated by IoT devices grows, edge computing is becoming more important, allowing data processing to occur closer to the data source.
-
Augmented Analytics: Augmented analytics uses machine learning and AI to assist with data preparation, insight generation, and insight explanation, making analytics more accessible to a broader audience.
-
Generative AI: While generative AI is sparking interest, it needs to deliver practical value to become a mainstay in data science and analytics.
Conclusion
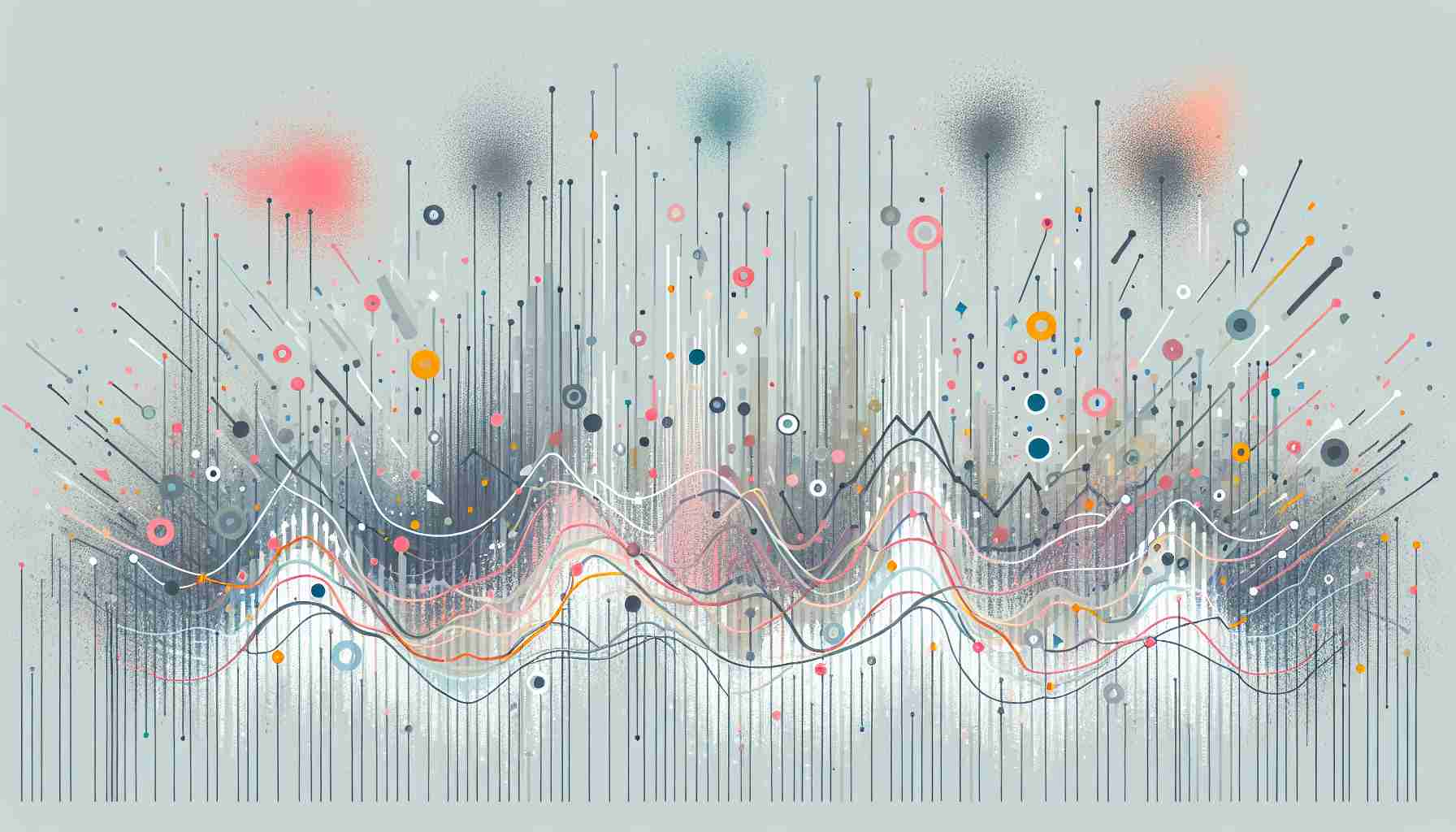
Data trends are a fundamental aspect of data analysis, providing the insights necessary for organizations to navigate an ever-changing landscape. As we continue to witness rapid technological advancements, the ability to identify and leverage these trends will become increasingly important. Businesses that stay abreast of the latest data analytics trends and incorporate them into their strategies will be well-positioned to thrive in the years to come.
FAQs on Data Trends
Q: What are data trends? A: Data trends are identifiable patterns, changes, or movements in data over time that can be used to predict future events or understand past performance.
Q: Why are data trends important? A: Data trends are important because they help businesses and organizations make informed decisions, anticipate market changes, and develop strategies based on empirical evidence.
Q: How are data trends identified? A: Data trends are identified using statistical analysis, data visualization tools, and platforms like Google Trends that analyze search query volumes to gauge public interest.
Q: What are some current data analytics trends? A: Some current trends include AI-powered data analytics, increased focus on data privacy and security, the rise of hybrid cloud solutions, the development of data fabric, edge computing, augmented analytics, and the potential of generative AI.
Q: How has the analysis of data trends evolved over time? A: The analysis of data trends has evolved with advancements in technology, particularly with the integration of AI and machine learning, which have made data analysis more accurate and insightful.
Sources
- Google Trends
- 7 Top Data Analytics Trends In 2024 - Exploding Topics
- Google Trends
- The Top Data and Analytics Trends for 2024 (That Aren't Generative ...
- Navigating the Data Landscape in 2024: 2 Key Trends to Watch
- 10 Data and Analytics Trends to Keep on Your Radar - Gartner
- Top Trends in Big Data for 2024 and Beyond | TechTarget
- Top 10 Data Analytics Trends You Must Know in 2024
- Top 10 Analytics & Business Intelligence Trends For 2024 - Datapine
- Five Key Trends in AI and Data Science for 2024
- Top Data Analytics Trends Shaping 2024 - Simplilearn.com