Decision Intelligence Harnessing Data for Smarter Decision-Making
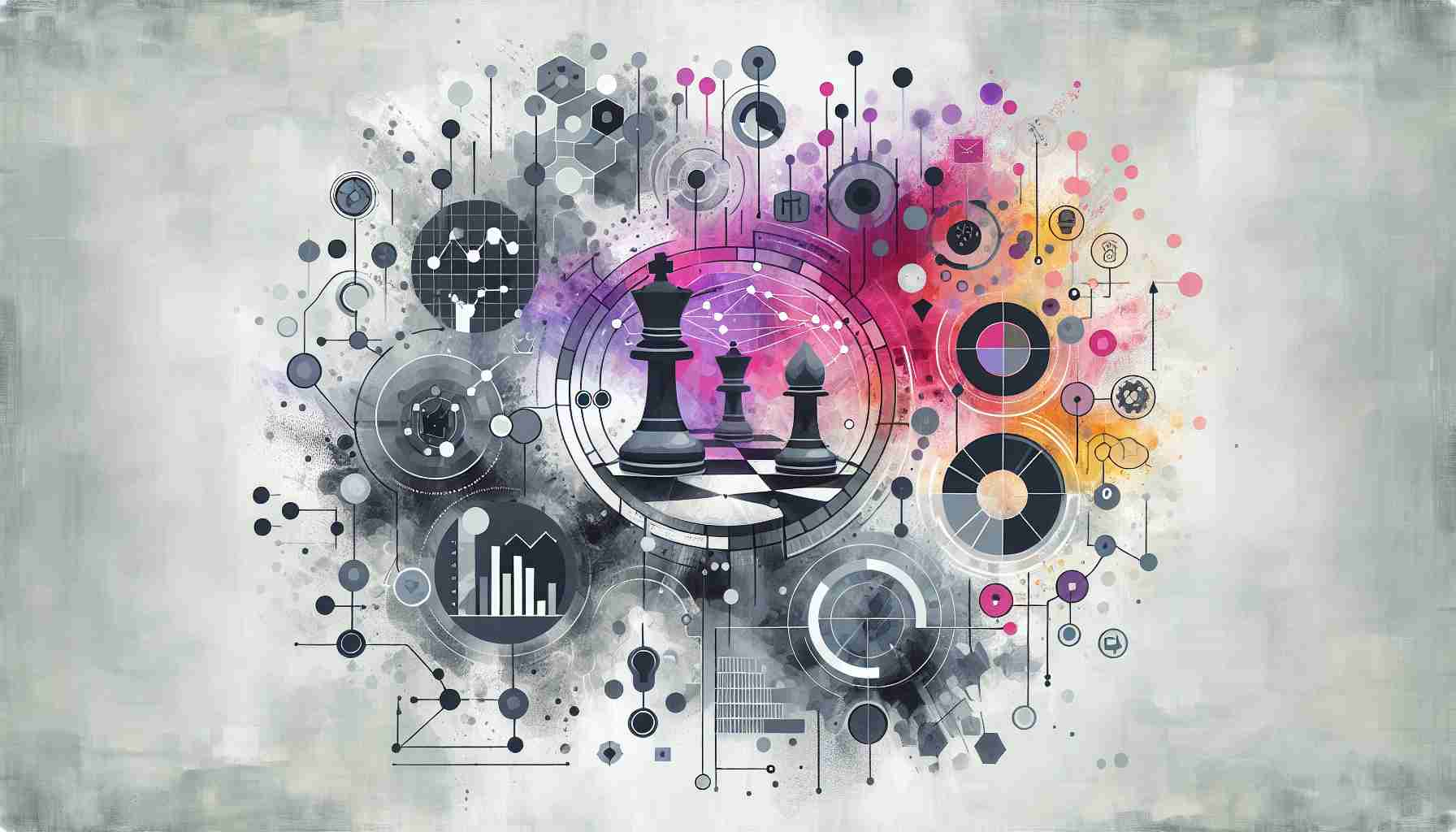
Decision Intelligence Briefly Summarized
- Decision intelligence is an interdisciplinary field that combines data science with theories from social science, decision theory, and managerial science.
- It provides a framework for organizational decision-making, leveraging AI technologies like machine learning and natural language processing.
- The core concept revolves around understanding the cause-and-effect relationship between actions and outcomes.
- Decision intelligence involves decision modeling, a visual language to represent the cause-and-effect chains.
- It is closely related to decision engineering, which focuses on improving decision-making processes, often with a strong data science component.
The world of data analysis has evolved dramatically with the advent of decision intelligence (DI), a field that is rapidly becoming indispensable in the realm of business and organizational strategy. At its heart, decision intelligence is about making informed decisions by understanding the intricate web of cause and effect that governs the outcomes of our actions.
What is Decision Intelligence?
Decision intelligence is an engineering discipline that augments data science with theory from social science, decision theory, and managerial science. It's a holistic approach to decision-making that not only considers the data at hand but also the human and organizational factors that influence the decision-making process. By integrating these diverse fields, decision intelligence aims to provide a comprehensive framework that helps organizations make better, more informed decisions.
The Components of Decision Intelligence
Data Science and AI Technologies
At the core of decision intelligence is the use of advanced data science techniques and AI technologies such as machine learning (ML), natural language processing (NLP), reasoning, and semantics. These technologies enable the analysis of large datasets to uncover patterns, predict outcomes, and generate insights that can inform decision-making.
Decision Theory and Social Science
Decision theory provides the mathematical and philosophical underpinnings of decision intelligence, offering models and principles on how decisions are made under uncertainty. Social science contributes an understanding of human behavior and organizational dynamics, which are crucial for implementing decisions effectively.
Managerial Science
Managerial science brings in the strategic aspect, focusing on how decisions align with an organization's goals and objectives. It provides the tools and processes for decision-making that are scalable and repeatable across different levels of an organization.
The Process of Decision Intelligence
The process of decision intelligence can be broken down into several key steps:
- Data Collection and Management: Gathering relevant data from various sources and ensuring its quality and accessibility.
- Insight Generation: Analyzing the data to uncover trends, correlations, and insights that can inform decisions.
- Decision Modeling: Using visual languages to represent the cause-and-effect relationships and potential decision paths.
- Decision Execution: Implementing the chosen course of action based on the insights and models developed.
- Monitoring and Tuning: Continuously tracking the outcomes of decisions and refining the decision-making process for better results.
Applications of Decision Intelligence
Decision intelligence can be applied across various domains, including but not limited to:
- Business Strategy: Enhancing competitive advantage by making data-driven strategic decisions.
- Operations: Optimizing supply chain management, inventory control, and production processes.
- Marketing: Tailoring marketing campaigns and customer engagement strategies based on predictive analytics.
- Finance: Managing risk and investment strategies by analyzing market trends and financial data.
- Human Resources: Improving hiring, retention, and employee development through data-informed HR practices.
The Future of Decision Intelligence
As organizations continue to generate and collect vast amounts of data, the role of decision intelligence will only grow in importance. It is poised to become a key driver of innovation, efficiency, and competitive advantage in the data-driven economy.
Conclusion
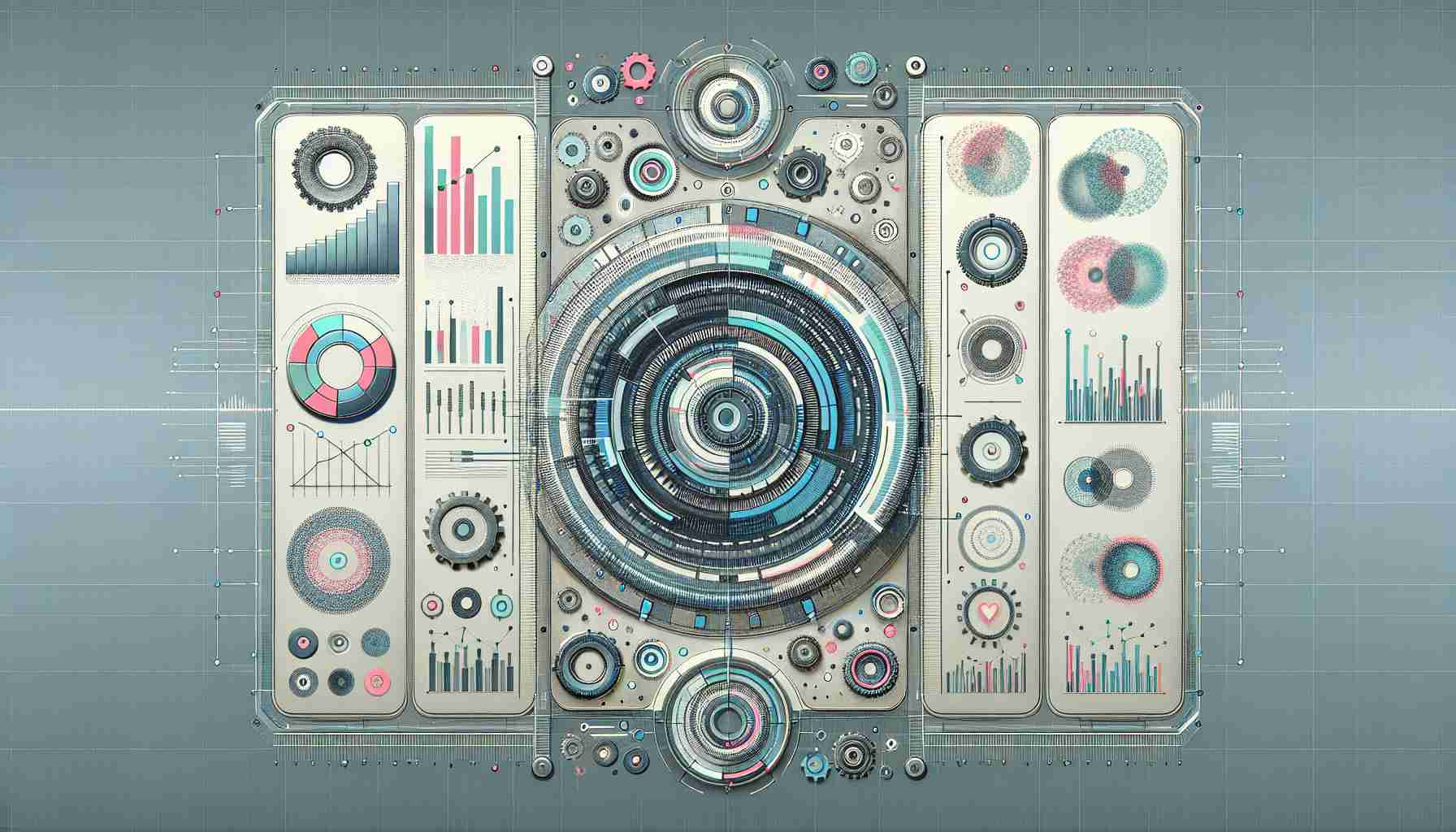
Decision intelligence represents a paradigm shift in how organizations approach decision-making. By leveraging the power of data science and AI, and integrating it with insights from social science, decision theory, and managerial science, decision intelligence offers a robust framework for navigating the complex landscape of modern business.
FAQs on Decision Intelligence
Q: How does decision intelligence differ from traditional data analysis? A: Traditional data analysis focuses on extracting insights from data, while decision intelligence goes a step further by applying these insights to make informed decisions, considering the broader context of human behavior and organizational goals.
Q: Can decision intelligence replace human decision-makers? A: No, decision intelligence is designed to augment and support human decision-making, not replace it. It provides valuable insights and recommendations that can help decision-makers choose the best course of action.
Q: Is decision intelligence only for large organizations? A: While large organizations may have more resources to invest in decision intelligence, the principles and tools of DI can be scaled and adapted for businesses and organizations of any size.
Q: How does decision intelligence use machine learning? A: Machine learning is used within decision intelligence to analyze data, identify patterns, make predictions, and generate insights that can inform decision-making processes.
Q: What are the challenges of implementing decision intelligence? A: Challenges include ensuring data quality, integrating diverse data sources, aligning decision intelligence with organizational goals, and managing the cultural shift towards data-driven decision-making.
Sources
- Decision intelligence
- What is Decision Intelligence? - Peak AI
- What is Decision Intelligence Guide - Quantexa
- Decision intelligence - Wikipedia
- What is decision intelligence? | Cognyte
- Is Decision Intelligence The New AI? - Forbes
- Decision Intelligence: How Untapped Data Unlocks Smarter Analytics
- What Is Decision Intelligence? - Alteryx
- Introduction to Decision Intelligence | by Cassie Kozyrkov
- Decision Intelligence: What It Is and Why It Matters - Tellius