Machine Learning vs AI Understanding the Distinctions in Data Analysis
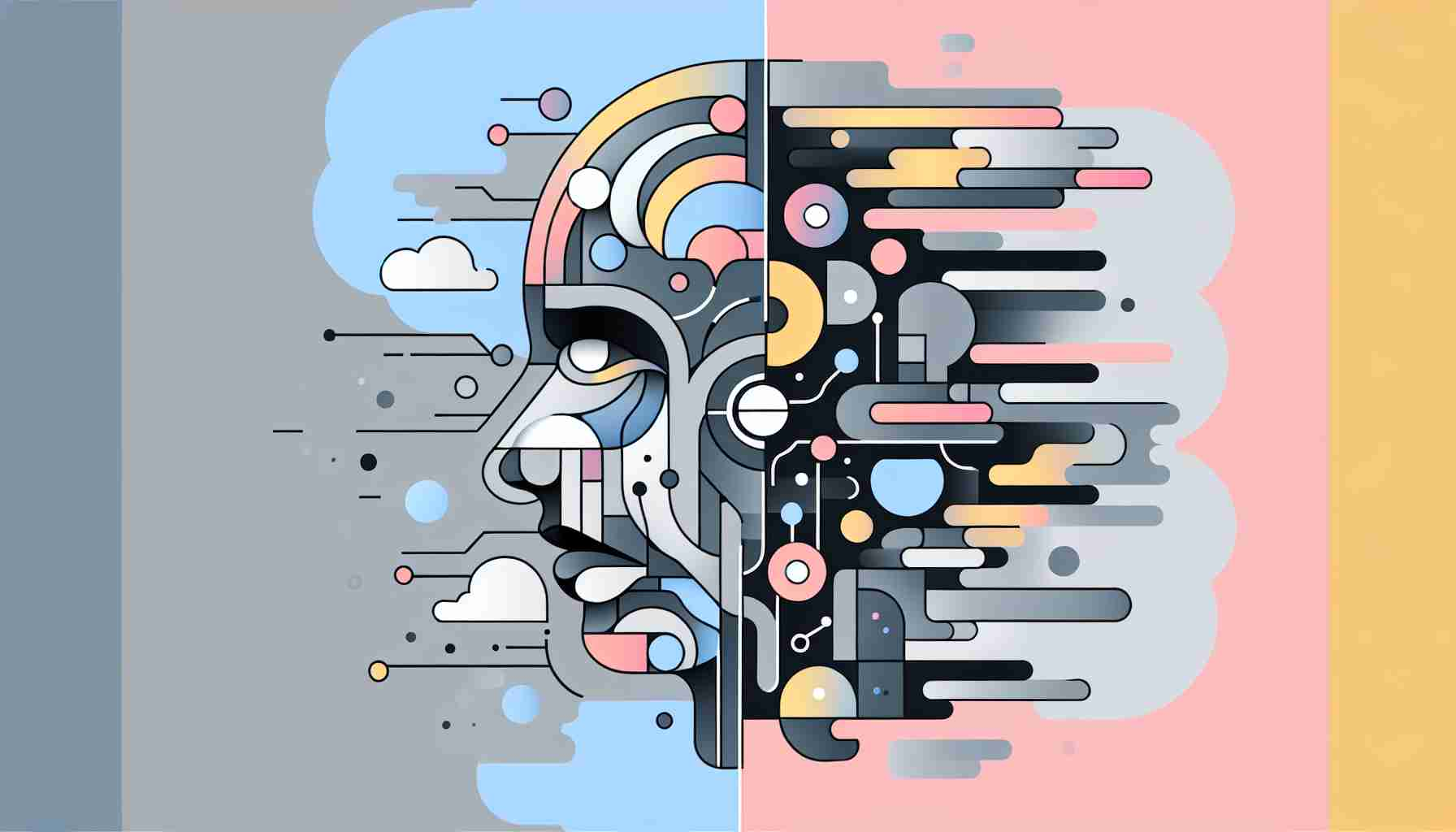
Machine Learning vs AI Briefly Summarized
- Artificial Intelligence (AI) is a broad field that encompasses machine intelligence, aiming to replicate human cognitive functions.
- Machine Learning (ML) is a subset of AI focused on algorithms that enable computers to learn from and make predictions or decisions based on data.
- AI includes various sub-fields such as reasoning, natural language processing, and robotics, while ML is primarily concerned with data-driven learning and pattern recognition.
- The development of AI and ML has led to significant advancements in industries like healthcare, finance, and autonomous vehicles.
- Ethical considerations and regulatory policies are increasingly important as AI and ML become more integrated into society.
Artificial Intelligence (AI) and Machine Learning (ML) are two of the most significant technological advancements in recent history, revolutionizing the way we analyze data and make decisions. While the terms are often used interchangeably, they have distinct meanings and implications. This article aims to demystify the concepts of AI and ML, explore their differences and uses, and highlight their impact on data analysis.
Introduction to AI and ML
Artificial Intelligence (AI) is the broader concept of machines being able to carry out tasks in a way that we would consider "smart". It's a field of computer science that aims to create systems capable of performing tasks that typically require human intelligence. These tasks include problem-solving, reasoning, understanding natural language, and recognizing patterns.
Machine Learning, on the other hand, is a subset of AI that involves the development of algorithms that can learn from and make predictions or decisions based on data. ML systems improve their performance over time as they are exposed to more data.
The Evolution of AI and ML
AI has a rich history that dates back to the mid-20th century. Alan Turing, often considered the father of computer science, was one of the first to explore the concept of machine intelligence. Since then, AI has gone through various cycles of high expectations followed by disappointment and reduced funding, known as "AI winters." However, the field has seen a resurgence, especially since the advent of deep learning and the transformer architecture in the 2010s, leading to what some call the "AI spring."
Machine Learning has similarly evolved, with significant advancements in algorithms and computational power. The introduction of neural networks and deep learning has particularly revolutionized the field, enabling ML systems to perform complex tasks with unprecedented accuracy.
AI vs ML: The Key Differences
While AI and ML are closely related, they are not synonymous. Here are some key distinctions:
- Scope: AI is a broader concept that includes ML. AI aims to mimic human intelligence and can include rule-based systems that do not learn from data. ML is specifically focused on learning from data and improving over time.
- Functionality: AI systems can perform a wide range of intelligent tasks, including those that do not involve learning. ML systems are designed to learn from data and make predictions or decisions based on that learning.
- Approach: AI can use a variety of approaches to achieve intelligent behavior, including handcrafted rules and logic. ML relies on statistical methods to find patterns in data.
Applications of AI and ML
AI and ML have a wide range of applications across various industries:
- Healthcare: AI is used for diagnostic systems, treatment planning, and personalized medicine. ML algorithms analyze medical data to predict patient outcomes and assist in diagnosis.
- Finance: AI is employed for fraud detection, risk management, and automated trading. ML models are used to predict stock market trends and customer behavior.
- Autonomous Vehicles: AI powers the decision-making systems of self-driving cars. ML is used to interpret sensor data and make real-time driving decisions.
Ethical Considerations and Future Directions
The integration of AI and ML into society raises important ethical questions. Issues such as privacy, bias, and accountability must be addressed to ensure that these technologies are used responsibly. Regulatory policies are being discussed to safeguard the benefits of AI and ML while minimizing their risks.
Conclusion
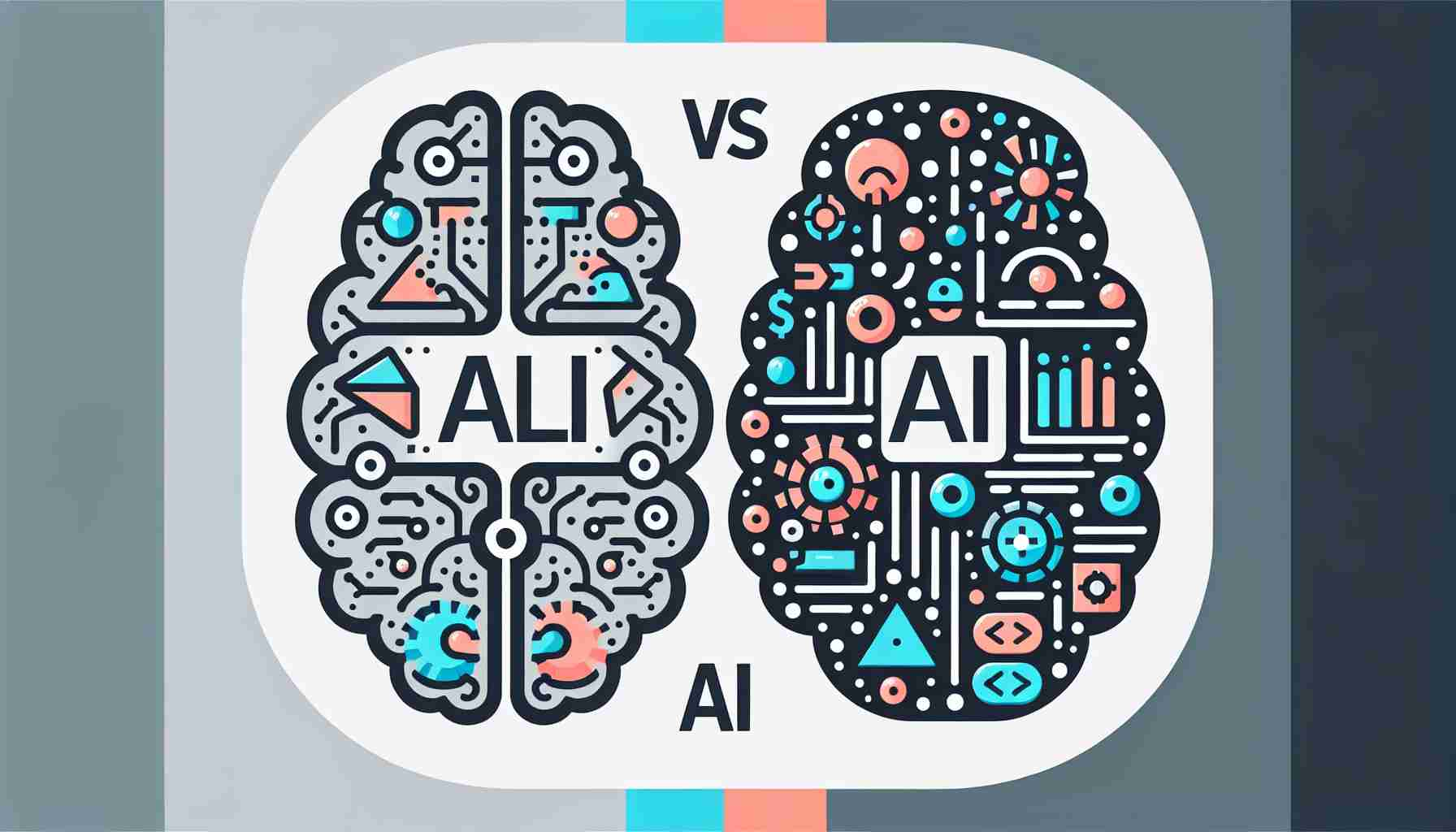
AI and ML are transformative technologies that have reshaped the landscape of data analysis and decision-making. Understanding the differences between AI and ML is crucial for leveraging their full potential and navigating the ethical challenges they present.
FAQs on Machine Learning vs AI
-
What is the main difference between AI and ML? AI is the broader concept of machines mimicking human intelligence, while ML is a subset of AI focused on algorithms that learn from data.
-
Can AI exist without ML? Yes, AI can exist without ML in the form of rule-based systems that do not learn from data but instead follow pre-programmed instructions.
-
Is Deep Learning the same as ML? Deep Learning is a subset of ML that uses neural networks with many layers to learn from large amounts of data.
-
How do AI and ML impact job markets? AI and ML are automating tasks and creating new job opportunities, but they also pose challenges for job displacement and require workforce reskilling.
-
What are some ethical concerns with AI and ML? Ethical concerns include data privacy, algorithmic bias, transparency, and the impact of automation on employment.
-
How can I start a career in AI or ML? A career in AI or ML typically requires knowledge in computer science, mathematics, and statistics, with specialized education or training in AI/ML concepts and technologies.
Sources
- Artificial intelligence
- Artificial Intelligence (AI) vs. Machine Learning | Columbia AI
- AI vs. Machine Learning: How Do They Differ? | Google Cloud
- What is the difference between AI and Machine learning? - Reddit
- AI vs Machine Learning - YouTube
- Machine Learning vs. AI: Differences, Uses, and Benefits | Coursera
- AI vs. Machine Learning vs. Deep Learning vs. Neural Networks - IBM
- Machine Learning vs Artificial Intelligence: What's the Difference?
- Deep Learning vs. Machine Learning: A Beginner's Guide - Coursera
- Artificial intelligence (AI) vs. machine learning (ML) - Microsoft Azure