Streaming Data The Pulse of Real-Time Analytics
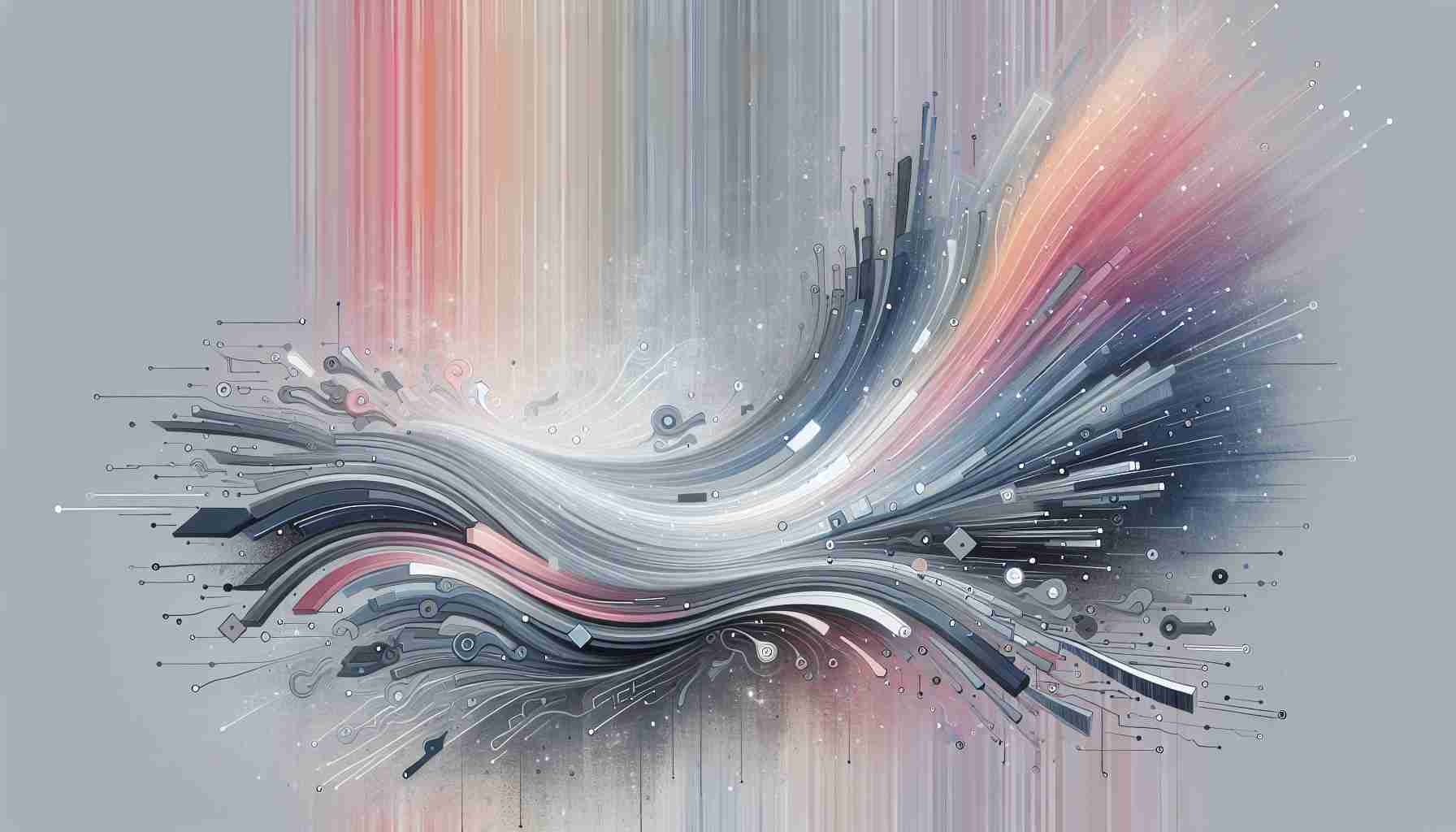
Streaming Data Briefly Summarized
- Streaming data is a continuous flow of data generated from various sources that is processed incrementally.
- It is essential for real-time analytics and is often associated with big data, requiring stream processing techniques.
- Concept drift is a phenomenon where the properties of the data stream can change over time.
- Unlike data lakes, which store large volumes of data, streaming data is analyzed on-the-fly without the need for storage.
- Streaming data is pivotal for businesses to analyze customer behavior in real-time and make immediate, data-driven decisions.
Streaming data has become an integral part of modern data analysis, especially in the era of big data. As businesses and organizations strive to make more informed decisions faster than ever before, the ability to process and analyze data in real time has become crucial. This article delves into the world of streaming data, exploring its definition, importance, and applications in various industries.
What is Streaming Data?
Streaming data is a dynamic, continuous sequence of data generated by numerous sources at high velocity. This data is characterized by its incessant flow, requiring different processing methods than static datasets. Streaming data is not stored in its entirety but is processed incrementally as it arrives, enabling immediate insights and actions.
The concept of streaming data is often linked to the term "big data" due to the volume, velocity, and variety of the information involved. With the proliferation of internet-connected devices, social media platforms, and various digital sensors, the amount of data generated every second is colossal. This data includes everything from financial transactions and social media posts to telemetry from IoT devices and logs from web servers.
Why is Streaming Data Important?
The importance of streaming data lies in its timeliness. In many scenarios, the value of data diminishes as it ages. For instance, detecting fraud as it happens can prevent significant financial loss, while understanding customer preferences in real-time can lead to better service and increased sales. Streaming data allows organizations to react to events as they occur, rather than after the fact.
Moreover, streaming data is crucial for predictive analytics. By analyzing data streams, businesses can forecast trends, anticipate demands, and prepare for future events with greater accuracy. This proactive approach can lead to competitive advantages in various sectors, from retail to finance to healthcare.
Processing Streaming Data
Processing streaming data requires a different approach compared to batch processing, where data is collected over a period and processed in large chunks. Stream processing involves continuously ingesting data as it arrives and providing outputs almost instantaneously. This is achieved through stream processing engines and frameworks such as Apache Kafka, Apache Flink, and Amazon Kinesis.
These technologies enable the handling of data that is in constant motion, allowing for complex operations such as filtering, aggregating, and windowing. Windowing is a technique where data is processed in finite chunks within a specified time frame, which is essential for managing the unbounded nature of data streams.
Challenges of Streaming Data
One of the main challenges of streaming data is dealing with concept drift. As the properties of the data stream change over time, the models and algorithms used to process and analyze the data must adapt accordingly. This requires sophisticated machine learning techniques that can evolve with the data.
Another challenge is ensuring data quality and consistency. With data streaming in from various sources, there is a risk of duplicates, missing information, or errors. Effective stream processing must include mechanisms to clean and validate data in real time.
Applications of Streaming Data
Streaming data has a wide range of applications across different industries. In the financial sector, it is used for high-frequency trading and fraud detection. In marketing, it enables real-time personalization and targeted advertising. In logistics, streaming data can optimize supply chains by providing up-to-the-minute information on inventory levels and delivery statuses.
The healthcare industry also benefits from streaming data by monitoring patient vitals in real time, which can be life-saving in critical care situations. In the realm of smart cities, streaming data from sensors can help manage traffic flows, reduce energy consumption, and enhance public safety.
Conclusion
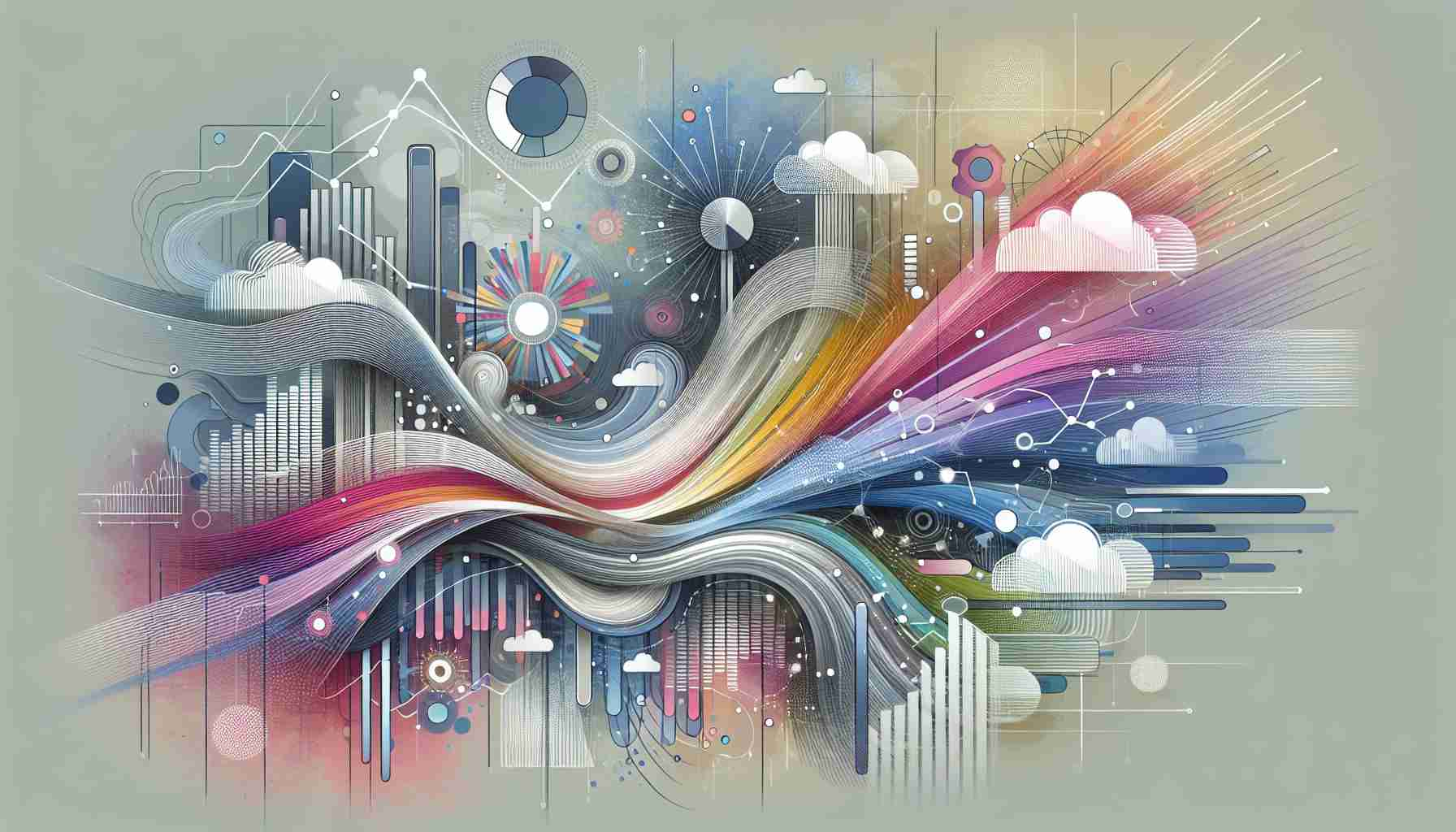
The ability to process and analyze streaming data is no longer a luxury but a necessity for businesses looking to thrive in a data-driven world. As technology continues to advance, the tools and techniques for streaming data analysis will become more sophisticated, enabling even deeper insights and more immediate actions. Understanding and harnessing the power of streaming data is key to unlocking the potential of real-time analytics and staying ahead in the competitive landscape.
FAQs on Streaming Data
What is streaming data? Streaming data is the continuous flow of data generated by various sources, processed in real time without the need for storage.
Why is streaming data important? It allows for immediate analysis and decision-making, which is crucial for applications such as fraud detection, real-time personalization, and predictive analytics.
How is streaming data processed? It is processed using stream processing engines and frameworks that handle continuous data ingestion and provide real-time outputs.
What are the challenges of streaming data? Challenges include dealing with concept drift, ensuring data quality, and managing the high velocity and volume of data.
What industries benefit from streaming data? Many industries benefit, including finance, marketing, logistics, healthcare, and smart city initiatives.
Sources
- Streaming data
- Streaming data - Wikipedia
- Data Streaming: Benefits, Examples, and Use Cases - Confluent
- Data Streaming: A Complete Introduction - Splunk
- What is Real-Time Data Streaming? - AWS
- What is Streaming Data? Definition & Best Practices - Qlik
- Streaming Data - Manning Publications
- What Is Data Streaming? | Definition from TechTarget
- Understanding Data Streaming and Its Significance for ... - Spotfire
- What is Streaming Analytics? - Google Cloud