Supply Chain Analytics Harnessing Data for Enhanced Operational Efficiency
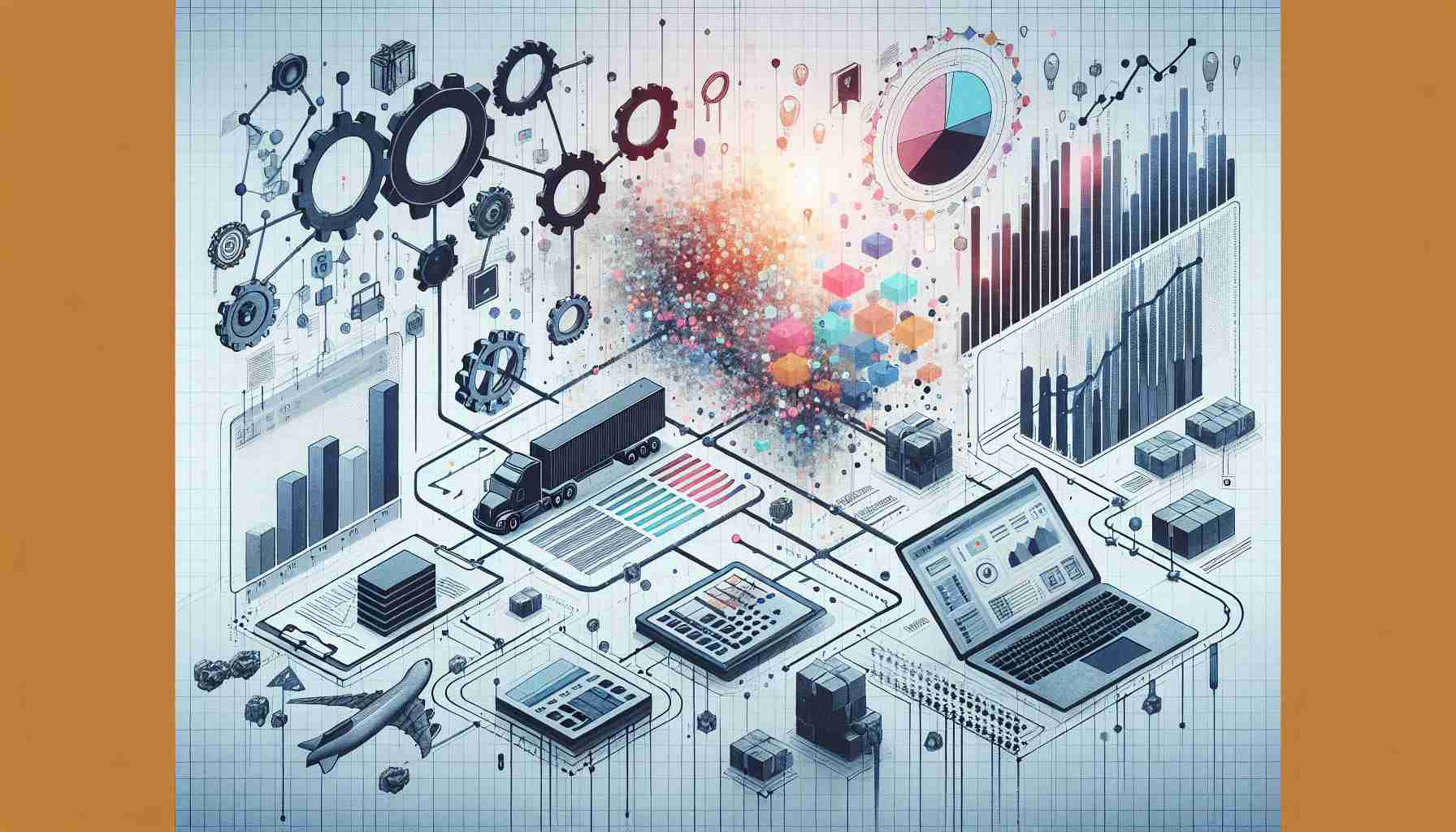
Supply Chain Analytics Briefly Summarized
- Supply Chain Analytics involves the strategic use of data to optimize the end-to-end supply chain process.
- It encompasses descriptive, predictive, and prescriptive analytics to understand, forecast, and improve supply chain operations.
- Analytics can identify and predict risks, as well as uncover opportunities for efficiency gains and cost savings.
- The integration of analytics into supply chain management supports data-driven decision-making and enhances competitiveness.
- Technological advancements such as AI and machine learning are increasingly pivotal in analyzing complex supply chain data.
The modern business landscape is characterized by a relentless quest for efficiency, cost reduction, and customer satisfaction. In this context, supply chain analytics emerges as a critical tool for organizations looking to gain a competitive edge. By leveraging data, businesses can streamline operations, predict future trends, and make informed decisions that ultimately lead to improved performance and profitability.
Introduction to Supply Chain Analytics
Supply chain analytics is the application of data analysis tools and techniques to improve the efficiency and effectiveness of supply chain processes. It involves collecting and analyzing data from various sources within the supply chain, including suppliers, manufacturers, distributors, and retailers. The insights gained from this analysis can help businesses to optimize inventory levels, enhance delivery performance, reduce costs, and improve overall supply chain agility.
The Role of Analytics in Supply Chain Management
Supply chain management (SCM) is a complex field that involves coordinating and optimizing a network of interconnected businesses that work together to provide products or services to the end customer. Analytics plays a crucial role in SCM by providing the insights needed to make strategic decisions. It helps in identifying bottlenecks, forecasting demand, managing inventory, and ensuring that the supply chain is resilient and responsive to changes in the market.
Types of Supply Chain Analytics
Supply chain analytics can be broadly categorized into three types:
-
Descriptive Analytics: This type of analytics focuses on what has happened in the past within the supply chain. It uses data to describe trends and relationships, such as supply chain performance or a warehouse's inventory levels.
-
Predictive Analytics: Predictive analytics uses historical data to forecast future events. It can identify known risks and help to predict future risks by spotting patterns and trends throughout the supply chain.
-
Prescriptive Analytics: Prescriptive analytics goes a step further by not only predicting what might happen but also suggesting actions that can be taken to influence the outcome. It involves the use of optimization and simulation algorithms to advise on possible outcomes and answer "what if" questions.
Implementing Supply Chain Analytics
Implementing supply chain analytics requires a strategic approach that includes the following steps:
- Data Collection: Gathering data from various sources within the supply chain, including internal systems and external partners.
- Data Integration: Combining data from multiple systems to create a unified view of the supply chain.
- Analysis: Applying statistical models and machine learning algorithms to analyze the integrated data.
- Insight Generation: Translating the results of the analysis into actionable insights.
- Decision Making: Using the insights to make informed decisions about the supply chain.
Challenges and Considerations
While supply chain analytics can provide numerous benefits, there are challenges that organizations must consider:
- Data Quality: Poor data quality can lead to inaccurate analysis and misguided decisions.
- Complexity: The supply chain can be incredibly complex, making it difficult to identify which data to analyze and how to interpret the results.
- Change Management: Implementing analytics-driven changes in the supply chain can require significant organizational adjustments.
- Security and Privacy: Ensuring the security and privacy of supply chain data is paramount, especially when dealing with sensitive or proprietary information.
The Future of Supply Chain Analytics
The future of supply chain analytics is closely tied to advancements in technology. Artificial intelligence (AI) and machine learning are becoming increasingly important in analyzing complex data sets and providing deeper insights. The Internet of Things (IoT) is also playing a role by providing real-time data from various points in the supply chain.
Conclusion
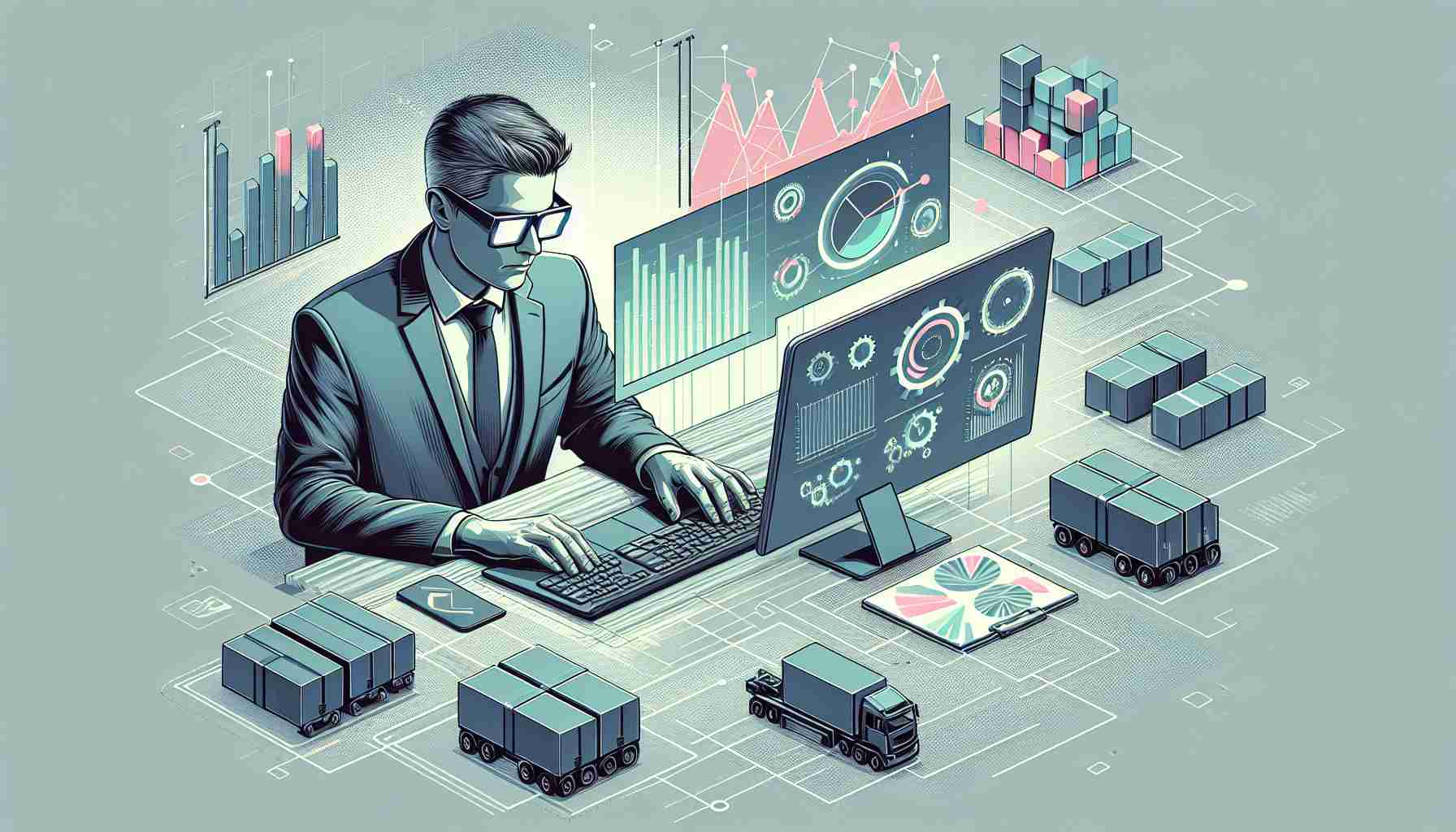
Supply chain analytics is a powerful tool that can transform the way organizations manage their supply chains. By harnessing the power of data, businesses can improve efficiency, reduce costs, and respond more effectively to market demands. As technology continues to evolve, the potential for supply chain analytics to drive innovation and competitive advantage will only increase.
FAQs on Supply Chain Analytics
Q: What is supply chain analytics? A: Supply chain analytics is the process of using data analysis techniques to improve the efficiency and effectiveness of the supply chain.
Q: Why is supply chain analytics important? A: It is important because it helps organizations to optimize their operations, reduce costs, forecast demand, and improve customer satisfaction.
Q: What are the main types of supply chain analytics? A: The main types are descriptive, predictive, and prescriptive analytics.
Q: How does supply chain analytics improve decision-making? A: It provides data-driven insights that help businesses to make informed decisions about their supply chain operations.
Q: What are some challenges associated with supply chain analytics? A: Challenges include ensuring data quality, managing complexity, implementing organizational changes, and maintaining data security and privacy.
Sources
- Business analytics
- What is supply chain analytics? - IBM
- Supply Chain Analytics: What It Is, Why It Matters, and More | Coursera
- MITx: Supply Chain Analytics | edX
- What is Supply Chain Analytics? Benefits & Best Practices - Qlik
- Supply Chain Analytics Explained: How it Works & Use Cases
- What is Supply Chain Analytics? - Towards Data Science
- The big-supply-chain analytics landscape (Part 1) - McKinsey
- Master of Supply Chain Analytics | Rutgers Business School
- What is Supply Chain Analytics and Why is It Important? - TechTarget
- What is Supply Chain Analytics? - YouTube